GitHub - ccxt/ccxt: cryptocurrency trading API with support for more than 100 bitcoin/altcoin exchanges 虽然ccxt本身不是一个完整的量化交易系统,而是一个交易所API接口的统一库,支持超过100个加密货币交易所。它为开发者提供了一套标准化的接口来交互各大交易所,非常适合构建自定义的量化交易系统。
GitHub - hummingbot/hummingbot: Open source software that helps you create and deploy high-frequency crypto trading bots Hummingbot是一个开源的算法交易机器人,专为加密货币市场设计。它支持多种交易策略,如做市、套利和跟随交易等,并且能够与众多主流交易所集成。
GitHub - DeviaVir/zenbot:Zenbot 是一个使用 Node.js 和 MongoDB 的命令行加密货币交易机器人。
GitHub - askmike/gekko:用节点编写的比特币交易机器人 - https://gekko.wizb.it/
GitHub - scrtlabs/catalyst: An Algorithmic Trading Library for Crypto-Assets in Python
CCXT(Cryptocurrency Exchange Trading Library)是一个非常受欢迎且功能强大的开源交易库,旨在为开发者提供一个统一的接口来接入全球范围内的多个加密货币交易所。以下是关于CCXT的详细信息:
- 目的:CCXT的主要目标是简化加密货币交易的开发流程,使得获取市场数据、下单交易、账户管理等操作变得简单易行。它通过提供一个统一的API接口,使得开发者无需为每个交易所单独编写适配代码,大大节省了开发时间和成本。
- 支持语言:CCXT目前支持Python、JavaScript和PHP三种主流编程语言,这意味着无论你使用哪种语言进行开发,都有可能通过CCXT与加密货币交易所进行交互。
- 交易所覆盖:它支持超过100家加密货币交易所,涵盖了大多数知名和一些小众的交易平台,而且这个列表还在不断更新中,以适应快速变化的加密货币市场。
- 功能特性:CCXT不仅支持市场数据的获取(如行情、订单薄、交易历史),还支持交易操作(如下单、取消订单、查询账户状态、资金管理等)。此外,它还具备API密钥管理、错误处理和异常报告等功能,有助于开发者安全高效地进行交易。
- 统一接口:CCXT为所有支持的交易所提供了一致的API调用方式,这意味着一旦熟悉了CCXT的使用,你可以轻松切换到任何它支持的交易所,无需重新学习每个交易所特定的API。
- 高级功能:除了基础的交易功能,CCXT还支持更高级的交易策略,如市场和限价订单、止盈止损订单等。
- 社区与文档:CCXT拥有活跃的开发者社区,提供了丰富的文档、示例代码和教程,帮助开发者快速上手并解决问题。
- 开源与可扩展:作为开源项目,CCXT鼓励社区贡献,你可以根据需要修改或扩展其功能,满足特定的交易需求。
- 量化交易:是CCXT最广泛应用的场景之一,它为量化交易者提供了一个强大的工具,可以快速开发和测试交易策略。
- 数据收集与分析:许多数据科学家和研究人员使用CCXT来收集加密货币市场数据,进行市场分析和预测。
1
2
| import ccxt
print(ccxt.exchanges) # print a list of all available exchange classes
|
该库在 Python 3.7.0+ 中支持具有 asyncio 和 async/await 的并发异步模式
1
| import ccxt.async_support as ccxt # link against the asynchronous version of ccxt
|
利用ccxt的公共 API 获取binance的ETH_USDT市场数据
1
2
3
4
5
6
7
8
9
10
11
12
13
14
15
16
17
18
19
20
21
22
23
24
25
26
27
28
29
30
31
32
33
34
35
36
37
38
| # 导入ccxt库
import ccxt
##无代理初始化Binance交易所对象 binance = ccxt.binance()
# 代理服务器地址和端口
proxy = 'http://127.0.0.1:10819'
# 初始化Binance交易所对象,并设置代理 # 初始化Binance交易所对象,这里我们不提供API密钥和私钥,因为我们只是获取公共数据
binance = ccxt.binance({
'proxies': {
'http': proxy,
'https': proxy,
}
})
# 加载ETH/USDT市场数据
markets = binance.load_markets()
# 获取ETH/USDT的市场标识符,用于后续请求
market_symbol = binance.market('ETH/USDT')['symbol']
# 获取ETH/USDT的最新行情信息(ticker)
ticker = binance.fetch_ticker(market_symbol)
# 打印ETH/USDT的最新价格
print(f"ETH/USDT 最新价格: {ticker['last']}")
# 获取ETH/USDT的订单簿
order_book = binance.fetch_order_book(market_symbol)
# 打印ETH/USDT订单簿的前5个卖单和买单
print("ETH/USDT 订单簿 - 前5个卖单:")
for ask in order_book['asks'][:5]:
print(f"价格: {ask[0]}, 数量: {ask[1]}")
print("\nETH/USDT 订单簿 - 前5个买单:")
for bid in order_book['bids'][:5]:
print(f"价格: {bid[0]}, 数量: {bid[1]}")
|
利用ccxt的公共 API 获取binance的ETH_USDT 下面的信息 ,每个写成一个函数。需要获取的东西:
1
2
3
4
5
6
7
8
9
10
11
12
13
14
15
16
17
18
19
20
21
22
23
24
25
26
27
28
29
30
31
32
33
34
35
36
37
38
39
40
| """
instruments/trading pairs
price feeds (exchange rates)
order books
trade history
tickers
OHLC(V) for charting
"""
def get_exchange_info():
"""获取交易所信息,包括所有交易对"""
return binance.load_markets()
def get_ticker(symbol='ETH/USDT'):
"""获取指定交易对的最新价格信息"""
return binance.fetch_ticker(symbol)
def get_order_book(symbol='ETH/USDT', limit=10):
"""获取指定交易对的订单簿,默认显示前10个深度"""
return binance.fetch_order_book(symbol, limit)
def get_trade_history(symbol='ETH/USDT', limit=10):
"""获取指定交易对的最近交易历史,默认显示最近10条记录"""
return binance.fetch_trades(symbol, limit)
def get_ohlcv(symbol='ETH/USDT', timeframe='1h', since=None, limit=100):
"""
获取指定交易对的OHLCV数据,用于图表绘制。
timeframe: 时间周期,如'1m', '5m', '1h', '1d'等。
since: 获取OHLCV数据的起始时间戳(可选)。
limit: 返回的数据条数限制(默认100,最大值取决于交易所)。
"""
return binance.fetch_ohlcv(symbol, timeframe, since, limit)
def get_exchange_status():
"""获取交易所的状态信息"""
return binance.fetch_status()
# 获取ETH/USDT的最新价格
ticker = get_ticker('ETH/USDT')
print(ticker)
|
加快处理,优化代码:
1
2
3
4
5
6
7
8
9
10
11
12
13
14
15
16
17
18
19
20
21
22
23
24
25
26
27
28
29
30
31
32
33
34
35
36
37
38
39
40
41
42
43
44
| import asyncio
from ccxt.async_support import binance
import time
async def main():
start_time = time.time()
# 初始化Binance交易所对象
exchange = binance({
'enableRateLimit': True, # 遵守ccxt的速率限制
})
# 加载ETH/USDT市场数据
markets = await exchange.load_markets()
# 获取ETH/USDT的市场标识符,用于后续请求
market_symbol = exchange.market('ETH/USDT')['symbol']
# 异步获取ETH/USDT的最新行情信息(ticker)
ticker = await exchange.fetch_ticker(market_symbol)
print(f"ETH/USDT 最新价格: {ticker['last']}")
# 异步获取ETH/USDT的订单簿
order_book = await exchange.fetch_order_book(market_symbol)
# 打印ETH/USDT订单簿的前5个卖单和买单
print("ETH/USDT 订单簿 - 前5个卖单:")
for ask in order_book['asks'][:5]:
print(f"价格: {ask[0]}, 数量: {ask[1]}")
print("\nETH/USDT 订单簿 - 前5个买单:")
for bid in order_book['bids'][:5]:
print(f"价格: {bid[0]}, 数量: {bid[1]}")
# 注意:下方的函数定义未修改,因为它们应该在异步上下文中被调用或重写为异步函数
# 关闭交易所连接
await exchange.close()
end_time = time.time()
print(f"执行时间:{end_time - start_time} 秒")
# 运行异步主函数
asyncio.run(main())
|
1
2
3
4
5
6
7
8
9
10
11
12
13
14
15
16
| time python public-eth-async.py
ETH/USDT 最新价格: 2995.39
ETH/USDT 订单簿 - 前5个卖单:
价格: 2995.4, 数量: 6.7067
价格: 2995.44, 数量: 0.8203
价格: 2995.45, 数量: 1.3379
价格: 2995.5, 数量: 0.6699
价格: 2995.51, 数量: 0.0399
ETH/USDT 订单簿 - 前5个买单:
价格: 2995.39, 数量: 26.2894
价格: 2995.38, 数量: 2.8766
价格: 2995.35, 数量: 3.336
价格: 2995.34, 数量: 3.9316
价格: 2995.31, 数量: 0.0721
执行时间:2.6888670921325684 秒
|
1
| {'symbol': 'ETH/USDT', 'timestamp': 1715165592564, 'datetime': '2024-05-08T10:53:12.564Z', 'high': 3106.0, 'low': 2978.4, 'bid': 3005.99, 'bidVolume': 3.6381, 'ask': 3006.0, 'askVolume': 74.2106, 'vwap': 3036.26648151, 'open': 3101.46, 'close': 3006.0, 'last': 3006.0, 'previousClose': 3101.45, 'change': -95.46, 'percentage': -3.078, 'average': 3053.73, 'baseVolume': 275326.9396, 'quoteVolume': 835965958.163883, 'info': {'symbol': 'ETHUSDT', 'priceChange': '-95.46000000', 'priceChangePercent': '-3.078', 'weightedAvgPrice': '3036.26648151', 'prevClosePrice': '3101.45000000', 'lastPrice': '3006.00000000', 'lastQty': '1.08230000', 'bidPrice': '3005.99000000', 'bidQty': '3.63810000', 'askPrice': '3006.00000000', 'askQty': '74.21060000', 'openPrice': '3101.46000000', 'highPrice': '3106.00000000', 'lowPrice': '2978.40000000', 'volume': '275326.93960000', 'quoteVolume': '835965958.16388300', 'openTime': '1715079192564', 'closeTime': '1715165592564', 'firstId': '1416144644', 'lastId': '1416939198', 'count': '794555'}}
|
这行数据看起来像是加密货币交易所中针对以太坊(ETH)兑美元稳定币(USDT)交易对的市场快照信息。以下是具体字段的解释:
- symbol: 交易对标识符,这里是 ETH/USDT。
- timestamp: 市场快照的 Unix 时间戳(毫秒)。
- datetime: 相对应的 ISO 8601 格式的日期和时间。
- high: 在统计期内的最高交易价格。
- low: 在统计期内的最低交易价格。
- bid: 买方出价(买一价),即市场上愿意支付的最高价格。
- bidVolume: 与最高买价相对应的以太坊数量。
- ask: 卖方报价(卖一价),即市场上愿意接受的最低价格。
- askVolume: 与最低卖价相对应的以太坊数量。
- vwap: 成交量加权平均价格。
- open: 统计时间段开始时的交易价格。
- close: 统计时间段结束时的交易价格(也是最新的交易价格)。
- last: 最新成交价格。
- previousClose: 上一个统计时间段结束时的价格。
- change: 价格变化量(close - open)。
- percentage: 百分比格式的价格变化。
- average: 开盘价和收盘价的平均值。
- baseVolume: 在统计时间段内交易的以太坊数量。
- quoteVolume: 与 baseVolume 对应的 USDT 交易量。
- info: 包含原始交易信息的对象,与上述大多数字段重复。
此行数据提供了 ETH/USDT 交易对在一个特定时间段内的交易信息,如价格变动、交易量等重要市场指标。这对于交易者、分析师和投资者来说是有价值的信息,
下面代码改下从binance 平台进行, 利用 proxy = ‘http://127.0.0.1:10819’ API密钥和私钥. 处理的对象是 ETH_USDT 。并且支持卖出和限价买入。
1
2
3
4
5
6
7
8
9
10
11
12
13
14
15
16
17
18
19
20
21
22
23
24
25
26
27
28
29
30
31
32
33
34
35
36
37
38
39
40
41
42
43
44
45
46
47
48
49
50
51
52
53
54
55
| # coding=utf-8
import ccxt
# 代理服务器配置
proxy = 'http://127.0.0.1:10819'
# 初始化Binance交易所对象,设置API密钥、私钥及代理
exchange = ccxt.binance({
'apiKey': 'YOUR_BINANCE_API_KEY',
'secret': 'YOUR_BINANCE_SECRET',
'proxies': {
'http': proxy,
'https': proxy,
},
})
# 加载Binance的市场数据
try:
markets = exchange.load_markets()
except ccxt.NetworkError as e:
print(f"网络错误: {e}")
except Exception as e:
print(f"其他错误: {e}")
# 打印Binance的ID和市场数据
print(exchange.id, markets)
# 获取ETH/USDT的订单簿
try:
order_book = exchange.fetch_order_book('ETH/USDT')
print("ETH/USDT的订单簿:", order_book)
except Exception as e:
print(f"获取订单簿时出错: {e}")
# 获取ETH/USDT的最新价格
try:
ticker = exchange.fetch_ticker('ETH/USDT')
print("ETH/USDT的最新价格:", ticker['last'])
except Exception as e:
print(f"获取最新价格时出错: {e}")
# 获取ETH/USDT的交易历史
try:
trades = exchange.fetch_trades('ETH/USDT')
print("ETH/USDT的交易历史:", trades[:5]) # 只打印最近5笔交易
except Exception as e:
print(f"获取交易历史时出错: {e}")
# 注意: 以下为示例代码,实际执行交易操作前请确保已替换占位符为真实API密钥和私钥,
# 并且理解相关风险。这里不执行实际交易操作以避免误操作。
# 卖出一个ETH以市价换取美元
# print(exchange.id, exchange.create_market_sell_order('ETH/USDT', 1))
# 限价买入,支付2500美元购买1个ETH
# print(exchange.id, exchange.create_limit_buy_order('ETH/USDT', 1, 2500.00))
|
下面是对您提供的代码的逐行注释解释:
1
2
3
4
5
6
7
8
9
10
11
12
13
14
15
16
17
18
19
20
21
22
23
24
25
26
27
28
29
30
31
32
33
34
35
36
37
38
39
40
41
42
43
44
45
46
47
48
49
50
51
52
53
54
55
56
57
58
59
60
61
62
63
64
65
66
| # 指定文件编码为UTF-8
# coding=utf-8
# 导入ccxt库
import ccxt
# 初始化HitBTC交易所对象,并设置verbose模式(输出更多调试信息)
hitbtc = ccxt.hitbtc({'verbose': True})
# 初始化BitMEX交易所对象
bitmex = ccxt.bitmex()
# 初始化火币Pro(Huobi Pro)交易所对象
huobipro = ccxt.huobipro()
# 初始化Exmo交易所对象,设置API密钥和私钥
exmo = ccxt.exmo({
'apiKey': 'YOUR_PUBLIC_API_KEY',
'secret': 'YOUR_SECRET_PRIVATE_KEY',
})
# 初始化Kraken交易所对象,同样设置API密钥和私钥
kraken = ccxt.kraken({
'apiKey': 'YOUR_PUBLIC_API_KEY',
'secret': 'YOUR_SECRET_PRIVATE_KEY',
})
# 动态获取Binance交易所类并初始化对象
exchange_id = 'binance'
exchange_class = getattr(ccxt, exchange_id)
exchange = exchange_class({
'apiKey': 'YOUR_API_KEY',
'secret': 'YOUR_SECRET',
})
# 加载HitBTC的市场数据
hitbtc_markets = hitbtc.load_markets()
# 打印交易所ID和市场数据
print(hitbtc.id, hitbtc_markets)
# 同样打印BitMEX和火币Pro的ID及市场数据
print(bitmex.id, bitmex.load_markets())
print(huobipro.id, huobipro.load_markets())
# 获取HitBTC的某个交易对的订单簿
print(hitbtc.fetch_order_book(hitbtc.symbols[0]))
# 获取BitMEX的BTC/USD的行情信息
print(bitmex.fetch_ticker('BTC/USD'))
# 获取火币Pro的LTC/USDT的交易记录
print(huobipro.fetch_trades('LTC/USDT'))
# 打印Exmo交易所的账户余额
print(exmo.fetch_balance())
# 在Exmo上以市价单卖出1个比特币
print(exmo.id, exmo.create_market_sell_order('BTC/USD', 1))
# 在Exmo上发出限价买单,以2500欧元购买1个比特币
print(exmo.id, exmo.create_limit_buy_order('BTC/EUR', 1, 2500.00))
# 在Kraken交易所发出一个带有额外参数的市价买入订单
# 注意这里的额外参数('trading_agreement': 'agree')是交易所特定的
kraken.create_market_buy_order('BTC/USD', 1, {'trading_agreement': 'agree'})
|
这段代码展示了如何使用CCXT库初始化多个加密货币交易所的对象,加载市场数据,获取订单簿、行情、交易记录、账户余额,以及如何在Exmo和Kraken交易所上下单。请注意,在实际使用中,您需要将'YOUR_PUBLIC_API_KEY'
、'YOUR_SECRET_PRIVATE_KEY'
以及交易对等占位符替换为您自己在相应交易所的真实凭据和正确的交易对名称。
python-binance CCXT Binance Connector
币安Python API简明教程 (defiplot.com)
binancefuture https://testnet.binancefuture.com
lfzwyc@gmail.com tong81*K
1
2
3
4
| API Key
0b8e6d7f81c3e01224bde98a8444024462f55e5c52e6d9708a935a9048ea65d5
API Secret
aebe0a64108b49f159c43a78fd276c1087db2cd308e48440f79ed4c3f3a8fc37
|
https://testnet.binance.vision/ github Logged in as test user 1715260059400324628
python-binance 使用ok
Save these values right now. They won’t be shown ever again!
1
2
3
4
5
6
7
8
9
10
11
12
13
14
15
16
17
18
19
20
|
Step 1: Generate the private key test-prv-key.pem. Do not share this file with anyone!
openssl genrsa -out test-prv-key.pem 2048
Step 2: Generate the public key test-pub-key.pem from the private key.
openssl rsa -in test-prv-key.pem -pubout -outform PEM -out test-pub-key.pem
得到
API Key: 0V1PQqJHSUp3lYMXLhgrEFZDTQbUnJTncCXxqtKPMtnxhpq26e8zEIg8QHjJRG1r
Public key: (for reference)
-----BEGIN PUBLIC KEY-----
MIIBIjANBgkqhkiG9w0BAQEFAAOCAQ8AMIIBCgKCAQEA6gz1j7V86iqwf3I9qwFS
Asbuo1HYHgY1iccANSnKtU9LCO6DEmJiT4xjgikDVNTHWzyOKXyDugFuSK1i6lE6
Y/FFyjlX3Tmk76ntHRIsVM385yQ2sUvK6F0e4dNlL7x4+lXXtbwPPoagF6mi/zTg
WrkKPE57ArL64Tm77G18GRbnvSIr4SfOLb2t5BoWuVQtXgmM3bzKbhLbT+hyTAVw
UgUMo9v2IkewZnqd77Q1VbWgy7WMle1RCgEhL5lO31cz7x9JUszps5LfcmfzrlWY
c5hNrCe94r+KM9aXpB9Eq6WgWDihtROgLlI0vCbJivvcorNwo/XWAOkdXUwG/gUy
qQIDAQAB
-----END PUBLIC KEY-----
|
买与卖
1
2
3
4
5
6
7
8
9
10
11
12
13
14
15
16
17
18
19
20
21
22
23
24
25
26
27
28
29
30
31
32
33
34
35
36
37
38
39
40
41
42
43
44
45
46
47
48
49
50
51
52
53
54
55
56
57
58
59
60
61
62
63
64
65
66
67
68
69
70
71
72
73
74
75
76
77
78
79
80
81
82
83
84
85
86
87
88
89
90
91
92
93
94
95
96
97
98
99
100
101
102
103
104
105
106
107
108
109
110
111
112
113
114
115
116
117
118
119
120
121
122
123
124
125
126
127
128
129
130
131
132
133
134
135
136
137
138
139
140
141
142
143
144
145
146
147
148
149
150
151
152
153
154
155
156
157
158
159
160
161
162
163
164
165
166
167
168
169
170
171
172
173
174
175
176
177
178
179
180
181
182
183
184
185
186
187
188
189
190
191
192
193
194
195
196
197
198
199
200
201
202
203
204
205
206
207
208
209
210
211
212
213
214
215
216
217
218
219
220
221
222
223
224
225
226
227
228
229
230
231
232
233
234
235
236
237
238
239
240
241
242
243
244
245
246
247
248
249
250
251
252
253
254
255
256
257
258
259
260
261
262
263
264
265
266
267
268
269
270
271
272
273
274
275
276
277
278
279
280
| ## https://testnet.binance.vision/
export binance_api="5nV9ChLDDCSgIPkNWpBrKJlKQOMdIleOjSdYp1gvvRCAaF5L9gZtRx20RM8CMxQ8"
export binance_secret="5xHbdWenlYBF8FwU4FtiKQfjcUl8EG3XmIpmuEmajptVoZe7tyxBMTZmblIrrO0K"
## ls https://testnet.binancefuture.com/
export binance_api="0b8e6d7f81c3e01224bde98a8444024462f55e5c52e6d9708a935a9048ea65d5"
export binance_secret="aebe0a64108b49f159c43a78fd276c1087db2cd308e48440f79ed4c3f3a8fc37"
import os
from binance.client import Client
# 初始化 init
api_key = os.environ.get('binance_api')
api_secret = os.environ.get('binance_secret')
client = Client(api_key, api_secret)
client.API_URL = 'https://testnet.binance.vision/api'
## 查看账号信息 余额 assets
# get balances for all assets & some account information
print(client.get_account())
# get balance for a specific asset only (BTC)
print(client.get_asset_balance(asset='BTC'))
print(client.get_asset_balance(asset='ETH'))
# get balances for futures account
print(client.futures_account_balance())
# get balances for margin account
print(client.get_margin_account())
## 查看最symbol新价格 get_symbol_ticker
# get latest price from Binance API
btc_price = client.get_symbol_ticker(symbol="BTCUSDT")
# print full output (dictionary)
print(btc_price)
print(btc_price["price"])
eth_price = client.get_symbol_ticker(symbol="ETHUSDT")
print(client.get_symbol_ticker(symbol="ETHUSDT")["price"])
## WebSocket 查看最symbol新价格
from time import sleep
from binance import ThreadedWebsocketManager
btc_price = {'error':False}
def btc_trade_history(msg):
if msg['e'] != 'error':
print(msg['c'])
btc_price['last'] = msg['c']
btc_price['bid'] = msg['b']
btc_price['last'] = msg['a']
btc_price['error'] = False
else:
btc_price['error'] = True
# init and start the WebSocket
bsm = ThreadedWebsocketManager()
bsm.start()
# subscribe to a stream
bsm.start_symbol_ticker_socket(callback=btc_trade_history, symbol='BTCUSDT')
bsm.start_symbol_ticker_socket(callback=btc_trade_history, symbol='ETHUSDT')
# stop websocket
bsm.stop()
help(ThreadedWebsocketManager)
start_symbol_ticker_socket
## CSV history 导出
# valid intervals - 1m, 3m, 5m, 15m, 30m, 1h, 2h, 4h, 6h, 8h, 12h, 1d, 3d, 1w, 1M
# get timestamp of earliest date data is available
timestamp = client._get_earliest_valid_timestamp('BTCUSDT', '1d')
print(timestamp)
# request historical candle (or klines) data
bars = client.get_historical_klines('BTCUSDT', '1d', timestamp, limit=1000)
# option 1 - save to file using json method
with open('btc_bars.json', 'w') as e:
json.dump(bars, e)
timestamp = client._get_earliest_valid_timestamp('BTCUSDT', '1d')
print(timestamp)
bars = client.get_historical_klines('BTCUSDT', '1d', timestamp, limit=1000)
# delete unwanted data - just keep date, open, high, low, close
for line in bars:
del line[5:]
# option 4 - create a Pandas DataFrame and export to CSV
btc_df = pd.DataFrame(bars, columns=['date', 'open', 'high', 'low', 'close'])
btc_df.set_index('date', inplace=True)
print(btc_df.head(30))
# export DataFrame to csv
btc_df.to_csv('btc_bars3.csv')
## 20 SMA
import btalib
import pandas as pd
# load DataFrame
btc_df = pd.read_csv('btc_bars3.csv', index_col=0)
btc_df.set_index('date', inplace=True)
btc_df.index = pd.to_datetime(btc_df.index, unit='ms')
# calculate 20 moving average using Pandas
btc_df['20sma'] = btc_df.close.rolling(20).mean()
print(btc_df.tail(5))
## order
print(client.get_symbol_ticker(symbol="ETHUSDT")["price"])
print(client.get_asset_balance(asset='ETH'))
# buy
## 限价买
buy_order_limit = client.create_order(
symbol='ETHUSDT',
side='BUY',
type='LIMIT',
timeInForce='GTC',
quantity=0.01,
price=3036)
## 现价买
buy_order = client.create_order(symbol='ETHUSDT', side='BUY', type='MARKET', quantity=0.01)
print(client.get_asset_balance(asset='ETH'))
print(client.get_symbol_ticker(symbol="ETHUSDT")["price"])
---
# 封装 限价买
import os
from binance.client import Client
from binance.enums import *
from binance.exceptions import BinanceAPIException, BinanceOrderException
api_key = os.environ.get('binance_api')
api_secret = os.environ.get('binance_secret')
client = Client(api_key, api_secret)
client.API_URL = 'https://testnet.binance.vision/api'
try:
buy_limit = client.create_order(
symbol='ETHUSDT',
side='BUY',
type='LIMIT',
timeInForce='GTC',
quantity=0.26,
price=3049)
except BinanceAPIException as e:
print(e)
except BinanceOrderException as e:
print(e)
## 查看自己的交易历史
import pandas as pd
trades_df = pd.DataFrame(client.get_my_trades(symbol='ETHUSDT')).sort_values(by='time', ascending=False)
print(trades_df.head(10).to_csv(index=False))
print(client.get_symbol_ticker(symbol="ETHUSDT")["price"], client.get_asset_balance(asset='ETH'), client.get_open_orders()) # 查看eth最新价格,余额,未完成的订单。
"""
import pandas as pd
# 获取特定交易对的成交历史
trades = client.get_my_trades(symbol='ETHUSDT')
#print(trades)
trades_df = pd.DataFrame(trades)
# 打印前20条记录 print(trades_df.head(20).to_csv(index=False))
trades_df_sorted = trades_df.sort_values(by='time', ascending=False)
# 获取最新的前10条记录
top_trades = trades_df_sorted.head(10)
# 输出最新前10条记录的CSV格式,不包括索引
print(top_trades.to_csv(index=False))
"""
---
#sell
## 限价卖 limit sell
sell_order_limit = client.create_order(
symbol='ETHUSDT',
side='sell',
type='LIMIT',
timeInForce='GTC',
quantity=0.01,
price=3045)
print(client.get_symbol_ticker(symbol="ETHUSDT")["price"]) # 获取eth 当前价格
print(client.get_asset_balance(asset='ETH')) # 获取eth余额(可自由交易的量)。
print(client.get_open_orders()) #获取未完成的订单
## 现价卖 market_sell
market_order = client.order_market_sell(symbol='ETHUSDT', quantity=0.01)
## ls order = client.create_order(symbol='ETHUSDT', side='SELL', type='MARKET', quantity=0.01)
---
##BTC 在5分内波动5%时,如何执行 ETH 交易?
v0
import os
from time import sleep
import pandas as pd
from binance import ThreadedWebsocketManager
from binance.client import Client
# init
api_key = os.environ.get('binance_api')
api_secret = os.environ.get('binance_secret')
client = Client(api_key, api_secret)
price = {'BTCUSDT': pd.DataFrame(columns=['date', 'price']), 'error': False}
def btc_pairs_trade(msg):
''' define how to process incoming WebSocket messages '''
if msg['e'] != 'error':
price['BTCUSDT'].loc[len(price['BTCUSDT'])] = [pd.Timestamp.now(), float(msg['c'])]
else:
price['error'] = True
# init and start the WebSocket
bsm = ThreadedWebsocketManager()
bsm.start()
bsm.start_symbol_ticker_socket(symbol='BTCUSDT', callback=btc_pairs_trade)
## main
while len(price['BTCUSDT']) == 0:
# wait for WebSocket to start streaming data
sleep(0.1)
sleep(300)
while True:
# error check to make sure WebSocket is working
if price['error']:
# stop and restart socket
bsm.stop()
sleep(2)
bsm.start()
price['error'] = False
else:
df = price['BTCUSDT']
start_time = df.date.iloc[-1] - pd.Timedelta(minutes=5)
df = df.loc[df.date >= start_time]
max_price = df.price.max()
min_price = df.price.min()
if df.price.iloc[-1] < max_price * 0.95:
try:
order = client.futures_create_order(symbol='ETHUSDT', side='SELL', type='MARKET', quantity=1)
break
except Exception as e:
print(e)
elif df.price.iloc[-1] > min_price * 1.05:
try:
order = client.futures_create_order(symbol='ETHUSDT', side='BUY', type='MARKET', quantity=1)
break
except Exception as e:
print(e)
sleep(0.1)
# properly stop and terminate WebSocket
bsm.stop()
|
Binance Connector 报错
https://testnet.binance.vision/
CCXT
1
2
3
4
5
6
7
8
9
10
| Private APIs allow the following:
manage personal account info
query account balances
trade by making market and limit orders
deposit and withdraw fiat and crypto funds
query personal orders
get ledger history
transfer funds between accounts
use merchant services
|
交易trading
取款withdrawals 不打勾
期货future 不打勾
restrict accesss to trusted ips only 选择
数据的准确度是否正确呢? 具体看这些【每日波动率 分钟时间段波动率 涨跌幅 成交量 价格阈值】
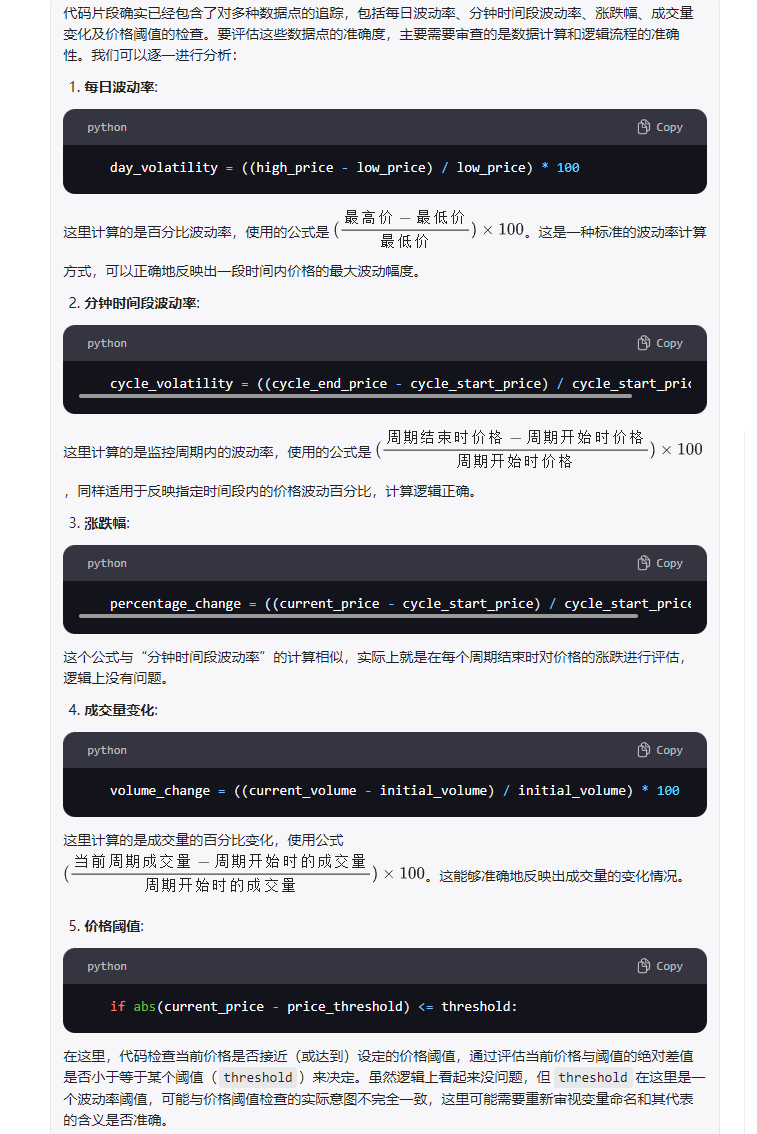
加密货币领域的量化交易系统是指应用数学模型、统计学方法以及算法策略,在加密货币市场上自动执行交易决策的过程。与传统金融市场上的量化交易类似,加密货币量化交易的核心目标是通过程序化交易策略来发现并利用市场机会,以实现盈利或风险管理。
加密货币市场的24小时不间断交易特性、高波动性以及全球性访问的便利性,为量化交易提供了丰富的应用场景。量化交易系统在加密货币市场中可能包含以下几个关键组成部分:
- 数据获取与处理:收集和清洗市场数据(如价格、交易量、订单薄等),这些数据是量化策略的基础。加密货币交易所通常通过API提供实时和历史数据。
- 策略开发:设计并测试交易策略,这些策略可以基于技术指标、统计套利、市场情绪、机器学习预测等多种方法。策略需要在历史数据上回测以验证其有效性。
- 执行引擎:将策略转化为可执行的交易指令,自动下单、平仓等操作,要求低延迟且准确无误。
- 风险管理:设定止损止盈规则,控制仓位大小,确保策略执行符合风险偏好,防止极端市场条件下的重大损失。
- 性能监控与优化:持续监控策略表现,根据市场变化调整策略参数,剔除失效策略,开发新的交易模型。
量化交易系统的优势在于能够迅速响应市场变化,减少人为情绪影响,实现全天候交易,以及通过多样化的策略组合来分散风险。然而,它也面临着模型过拟合、市场适应性、技术与监管风险等挑战。
使用CCXT库构建量化交易系统涉及几个核心步骤,下面是一个简化的流程,以帮助你开始构建自己的加密货币量化交易系统:
首先,确保安装了CCXT库。在Python中,你可以通过pip安装:
Bash
选择你想要交易的交易所,并初始化一个实例。这里以Binance为例:
Python
1
2
3
4
5
6
7
| 1import ccxt
2
3exchange = ccxt.binance({
4 'apiKey': 'YOUR_API_KEY',
5 'secret': 'YOUR_SECRET',
6 'enableRateLimit': True, # 遵守CCXT的速率限制
7})
|
加载交易所的市场数据,以便获取交易对的详细信息。
Python
1
| 1markets = exchange.load_markets()
|
根据市场数据和你的策略逻辑,设计交易信号生成器。这可能包括技术分析、基本面分析、机器学习模型等。
获取实时或历史数据,用于策略回测或实时交易决策。例如,获取最新的ticker信息:
Python
1
| 1ticker = exchange.fetch_ticker('BTC/USDT')
|
根据策略生成的信号,执行买入或卖出操作。例如,创建一个限价单:
Python
1
| 1order = exchange.create_order('BTC/USDT', 'limit', 'buy', 0.01, 50000)
|
实施风险管理策略,如设置止损、止盈订单,监控账户余额和持仓情况,确保交易活动符合预定的风险水平。
Python
1
| 1balance = exchange.fetch_balance()
|
在实际交易之前,使用历史数据对你的策略进行回测,评估其在不同市场条件下的表现。
部署你的策略到实时交易环境中,持续监控交易状态,根据市场变化和策略反馈进行调整。
记录交易活动,包括交易日志、性能报告,便于分析和审计。
量化交易系统的核心指标覆盖了多个方面,包括策略制定、风险管理、以及业绩评估等关键环节。以下是一些最为重要的量化交易系统指标:
- 收益率 (Return): 这是最直观的衡量标准,反映了策略在一段时间内的总盈利情况。通常会用年化收益率来比较不同时间跨度的绩效。
- 最大回撤 (Maximum Drawdown): 衡量资金从最高点跌落到最低点的幅度,用来评估策略在不利市场条件下的风险承受能力。较小的最大回撤意味着策略在下跌期间能较好地控制损失。
- 夏普比率 (Sharpe Ratio): 用来衡量每单位风险所带来的超额回报。较高的夏普比率表明策略在考虑风险后,能获得更好的回报。
- 索提诺比率 (Sortino Ratio): 类似于夏普比率,但只考虑下行风险,更适合评估对负面波动敏感的策略。
- 盈亏比 (Profit-Loss Ratio): 或称为赢率,即平均盈利交易的收益与平均亏损交易的损失之比,体现了策略盈利能力的一个方面。
- 胜率 (Win Rate): 成功交易次数占总交易次数的比例,反映策略选择正确方向的能力。
- 交易频率 (Trade Frequency): 指单位时间内执行交易的次数,影响成本和执行效率。
- 资金管理 (Position Sizing and Risk Management): 如凯利公式等,用于决定每次交易的资金分配,以优化风险调整后的收益。
- 市场适应性 (Robustness): 指策略在不同市场环境下的稳定性和适应能力,通常通过在不同时间段、不同市场数据上的回测来评估。
- 交易成本 (Transaction Costs): 包括手续费、滑点等,直接影响策略的净收益,必须在策略设计中予以考虑。
- 回测与外推误差 (Backtest vs. Out-of-Sample Performance): 回测表现与实际交易表现之间的差异,用于检验策略在未来市场的适用性。
- alpha与beta: alpha衡量策略相对于市场基准的超额收益,而beta则反映了策略相对市场的系统性风险。
构建量化交易系统时,综合考虑这些指标并优化策略参数,以达到风险可控、收益可期的目标。同时,不断监控和调整这些指标,以确保系统在不断变化的市场环境中保持竞争力。
在加密货币里面 尤其eth-usdt 推荐几个可靠的高收益率的交易策略
在加密货币交易中,特别是针对ETH/USDT这样的交易对,设计高收益率的交易策略时,需要结合技术分析、市场情绪、基本面分析及风险管理。请注意,任何策略都不能保证绝对的高收益,且加密市场波动极大,风险也相应较高。以下是一些常见的交易策略思路,但请在实际应用前做好充分的测试和风险评估:
- 趋势跟踪策略:
- 利用移动平均线(如50天和200天均线)来识别趋势,并在价格突破这些均线时开仓。
- 结合动量指标(如RSI或MACD)确认趋势强度,当指标显示超买或超卖时调整仓位。
- 网格交易:
- 在一定价格区间内设定多个买入和卖出价位,利用ETH/USDT的波动性自动低买高卖。
- 需要合理设置网格间距和资金分配,避免单边行情导致大量未成交订单。
- 对冲策略:
- 利用相关性较低的资产(如BTC或其他稳定币对)进行对冲,减少ETH价格波动的影响。
- 可以通过期权或期货市场进行更复杂的对冲操作。
- 套利策略:
- 在不同的交易所之间寻找ETH/USDT的价格差,迅速低买高卖赚取差价。
- 考虑到滑点和转账时间,此策略需要自动化工具和高速网络支持。
- 波段交易:
- 分析历史价格图表,识别支撑位和阻力位,区间内低吸高抛。
- 结合成交量和其他技术指标,判断波段转折点,适时入场出场。
- 算法交易:
- 使用智能算法根据预设规则自动执行交易,比如基于机器学习预测市场走势。
- 需要较强的技术背景和持续的数据优化。
重要提示:在实施上述任何策略之前,务必做好风险管理,包括但不限于设置止损点、控制仓位大小、定期评估策略效果并根据市场变化调整策略。此外,考虑到加密市场的高度波动性和不确定性,建议仅投入可承受损失的资金。在制定具体策略时,也可以参考最新的市场分析、新闻动态和技术指标,以做出更为精准的决策。
加密货币里面你觉得常用的,成熟的,盈利概率高的 趋势跟踪策略有哪些?
在加密货币市场中,趋势跟踪策略是一种广泛采用的方法,旨在识别并跟随市场的主要趋势,以实现盈利。以下是一些常用的、较为成熟且有较高盈利概率的趋势跟踪策略:
- 移动平均线(Moving Averages, MA)策略:
- 简单移动平均(SMA):计算一定周期内的平均价格,当短期均线上穿长期均线时视为买入信号,反之则卖出。
- 指数移动平均(EMA):赋予最近数据更多权重,反应更快,同样可以设置短期与长期均线交叉作为买卖信号。
- 布林带(Bollinger Bands):结合了移动平均线和标准差,当价格突破上轨可能预示上升趋势,突破下轨可能预示下降趋势。
- 相对强弱指数(Relative Strength Index, RSI):
- 通过比较资产的涨跌幅度来判断超买或超卖状态,通常RSI低于30被视为买入时机,高于70则是卖出时机。
- MACD(Moving Average Convergence Divergence):
- 利用快速和慢速移动平均线的差异来判断市场趋势变化,当MACD线(快速线)上穿信号线(慢速线)时为买入信号,反之则卖出。
- 动量指标(Momentum):
- 测量价格变动的速度,当价格上升速度加快,动量值增加,视为买入信号;下降则视为卖出信号。
- 斐波那契回撤与扩展:
- 用于确定潜在的支持和阻力水平,基于市场价格走势的自然比例,可以帮助识别趋势的延续或反转点。
- Ichimoku Cloud(一目均衡图):
- 提供了一套综合的市场分析框架,包括云层、转换线、基准线等,帮助识别趋势、支撑与阻力。
- 趋势线与通道:
- 通过手动绘制趋势线来识别价格的上升或下降趋势,通道策略则是在趋势内利用上下边界作为买卖依据。
- Donchian Channels(唐奇安通道):
- 基于过去一段时间内的最高价和最低价来定义上下边界,突破上边界视为买入,突破下边界视为卖出。
这些策略的成功在于正确识别并适应市场条件,以及合理设置参数和风险管理措施。没有任何单一策略能保证持续盈利,因此许多交易者会结合多种策略,并结合基本面分析和技术指标,同时严格控制风险,实施止损和资金管理规则。在实践中,持续监控市场动态、灵活调整策略,并进行适当的回测和实时测试是非常重要的。
给个实例,用ccxt 利用https://testnet.binance.vision/ 的 HMAC-SHA-256 Key registered API Key,Secret Key .构建量化交易系统。
selenium 与drissionpage 区别 哪个好。rpc 与rpa 区别 哪个好
Selenium 和 DrissionPage 都是用于自动化网页浏览器操作的工具库,但它们在设计理念、使用场景和功能特性方面存在一些区别。下面对这两个工具的主要特点进行比较,以帮助您根据实际需求选择合适的工具。
优点:
- 广泛的社区支持:Selenium 作为一个成熟的开源项目,拥有庞大的社区和广泛的资源,可以帮助解决各种问题。
- 跨浏览器兼容性:Selenium 支持几乎所有主流的浏览器,如 Chrome、Firefox、Edge 和 Safari,这使得它非常适合进行跨浏览器测试。
- 灵活性:Selenium 提供了对浏览器的底层控制,允许执行复杂的操作和集成。
缺点:
- 学习曲线:由于其功能强大,新手可能需要一段时间来学习如何有效地使用 Selenium。
- 资源消耗:Selenium 控制的是真实的浏览器实例,可能会占用较多的系统资源。
优点:
- 灵活的驱动管理:DrissionPage 可以灵活切换和管理无头浏览器和有头浏览器,使得网页操作更加高效。
- 简化操作:DrissionPage 旨在简化网页操作,如抓取和自动化任务,提供了更简单直观的 API,降低了使用的复杂度。
- 资源消耗较少:在无头模式下,DrissionPage 可以相比全功能浏览器占用更少的系统资源。
缺点:
- 社区和资源:相比于 Selenium,DrissionPage 的使用者较少,可能意味着寻找帮助和资源较为困难。
- 功能性:虽然对于大多数自动化任务来说已经足够,但 DrissionPage 在功能上可能不如 Selenium 灵活和全面。
选择哪个工具取决于您的具体需求:
- 如果您需要进行复杂的网页自动化,尤其是涉及多种浏览器的自动化测试,那么 Selenium 可能是更好的选择,因为它提供了广泛的支持和高度的灵活性。
- 如果您的项目主要是网页数据抓取和一些基本的网页操作,且希望操作简单、资源消耗低,那么 DrissionPage 可能更符合您的需求。
实际上,许多项目可能会根据不同的场景和需求,同时使用这两个工具来发挥各自的优势。
跟单交易|轻松复制专业交易者的交易策略,实现加密货币收益最大化|币安 (binance.com)
是的,这不正常。问题出在 trading_logic.py 中的深度分析计算逻辑。让我修改一下:
1
2
3
4
5
6
7
8
9
10
11
12
13
14
15
16
17
18
19
20
21
22
23
24
25
26
27
28
29
30
31
32
33
34
35
36
37
38
39
40
41
42
43
44
45
46
47
48
49
50
51
52
53
54
55
56
57
58
59
60
61
62
63
| def get_market_depth_analysis(self, df, symbol):
"""分析市场深度"""
symbol_df = df[df['symbol'] == symbol].copy()
if symbol_df.empty:
return {
'near': {'ratio': 0},
'mid': {'ratio': 0},
'far': {'ratio': 0}
}
current_price = float(symbol_df['current_price'].iloc[-1])
# 计算不同价格区间的订单累计量
price_ranges = {
'near': 0.001, # 0.1%
'mid': 0.005, # 0.5%
'far': 0.01 # 1%
}
depth_analysis = {}
for range_name, price_range in price_ranges.items():
try:
# 获取价格区间内的订单
bids_df = symbol_df[
(symbol_df['type'] == 'Bid') &
(symbol_df['price'].astype(float) >= current_price * (1 - price_range))
]
asks_df = symbol_df[
(symbol_df['type'] == 'Ask') &
(symbol_df['price'].astype(float) <= current_price * (1 + price_range))
]
# 计算买卖单总量
bids = bids_df['quantity'].sum() if not bids_df.empty else 0
asks = asks_df['quantity'].sum() if not asks_df.empty else 0
# 计算买卖比率
if asks == 0 and bids == 0:
ratio = 1.0 # 如果买卖单都为0,设置为1.0表示平衡
elif asks == 0:
ratio = 2.0 # 如果只有买单没有卖单,设置为2.0表示强烈买方压力
elif bids == 0:
ratio = 0.5 # 如果只有卖单没有买单,设置为0.5表示强烈卖方压力
else:
ratio = min(max(bids/asks, 0.5), 2.0) # 限制比率在0.5到2.0之间
depth_analysis[range_name] = {
'bid_volume': bids,
'ask_volume': asks,
'ratio': ratio
}
except Exception as e:
print(f"计算{symbol} {range_name}深度分析时发生错误: {e}")
depth_analysis[range_name] = {
'bid_volume': 0,
'ask_volume': 0,
'ratio': 1.0
}
return depth_analysis
|
主要改进:
- 分别获取买卖单数据后再计算总量
- 更合理的比率计算逻辑:
- 买卖单都为0时,比率为1.0(平衡)
- 只有买单时,比率为2.0(买方压力)
- 只有卖单时,比率为0.5(卖方压力)
- 正常情况下,比率限制在0.5到2.0之间
- 添加了更详细的错误信息输出
这样应该能得到更合理的深度分析结果。需要我解释更多细节吗?
为什么ETHUSDT和BTCUSDT的Depth Analysis 都显示为Depth Analysis: near:2 mid:2 far:2 这正常吗? 如果都为2 代表什么?
根据代码逻辑,当比率为 2.0 时表示在该价格区间内只有买单没有卖单(强烈买方压力)。让我们改进一下深度分析的逻辑:
1
2
3
4
5
6
7
8
9
10
11
12
13
14
15
16
17
18
19
20
21
22
23
24
25
26
27
28
29
30
31
32
33
34
35
36
37
38
39
40
41
42
43
44
45
46
47
48
49
50
51
52
53
54
55
56
57
58
59
60
61
62
63
64
65
66
67
68
69
70
71
72
73
74
75
76
77
78
79
80
81
| def get_market_depth_analysis(self, df, symbol):
"""分析市场深度"""
symbol_df = df[df['symbol'] == symbol].copy()
if symbol_df.empty:
return {
'near': {'ratio': 1.0, 'status': 'No Data'},
'mid': {'ratio': 1.0, 'status': 'No Data'},
'far': {'ratio': 1.0, 'status': 'No Data'}
}
current_price = float(symbol_df['current_price'].iloc[-1])
# 计算不同价格区间的订单累计量
price_ranges = {
'near': 0.001, # 0.1%
'mid': 0.005, # 0.5%
'far': 0.01 # 1%
}
depth_analysis = {}
for range_name, price_range in price_ranges.items():
try:
# 获取价格区间内的订单
bids_df = symbol_df[
(symbol_df['type'] == 'Bid') &
(symbol_df['price'].astype(float) >= current_price * (1 - price_range))
]
asks_df = symbol_df[
(symbol_df['type'] == 'Ask') &
(symbol_df['price'].astype(float) <= current_price * (1 + price_range))
]
# 计算买卖单总量
bids = bids_df['quantity'].sum() if not bids_df.empty else 0
asks = asks_df['quantity'].sum() if not asks_df.empty else 0
# 计算买卖比率和状态
if asks == 0 and bids == 0:
ratio = 1.0
status = 'No Orders'
elif asks == 0:
ratio = 2.0
status = 'Buy Only'
elif bids == 0:
ratio = 0.5
status = 'Sell Only'
else:
ratio = bids/asks
if ratio > 1.5:
status = 'Strong Buy'
elif ratio > 1.2:
status = 'Buy'
elif ratio < 0.67:
status = 'Strong Sell'
elif ratio < 0.83:
status = 'Sell'
else:
status = 'Neutral'
ratio = min(max(ratio, 0.5), 2.0) # 限制比率在0.5到2.0之间
depth_analysis[range_name] = {
'bid_volume': bids,
'ask_volume': asks,
'ratio': ratio,
'status': status
}
print(f"DEBUG: {symbol} {range_name} - Bids: {bids}, Asks: {asks}, Ratio: {ratio}, Status: {status}")
except Exception as e:
print(f"计算{symbol} {range_name}深度分析时发生错误: {e}")
depth_analysis[range_name] = {
'bid_volume': 0,
'ask_volume': 0,
'ratio': 1.0,
'status': 'Error'
}
return depth_analysis
|
然后修改 display.py 中的显示格式:
1
2
3
4
| # 在 create_figure 方法中修改深度分析文本的显示
depth_text = "Depth Analysis:\n" + "\n".join(
f"{k}: {v['ratio']:.2f} ({v['status']})" for k, v in depth_analysis.items()
)
|
这些改进:
- 添加了状态说明,更容易理解当前市场情况
- 添加了调试信息,帮助排查问题
- 保留了原始比率,只在显示时限制范围
- 状态说明:
- No Orders: 该价格区间无订单
- Buy Only: 只有买单
- Sell Only: 只有卖单
- Strong Buy: 买单量显著大于卖单量
- Buy: 买单量较大
- Strong Sell: 卖单量显著大于买单量
- Sell: 卖单量较大
- Neutral: 买卖相对平衡
这样我们就能更清楚地看到每个价格区间的具体情况。需要我解释更多细节吗?
让我解释一下趋势分析的参数和含义:
- B/A Ratio (Bid/Ask Ratio) 买卖比率:
- 6.88 表示买单量是卖单量的6.88倍
- 这是一个很强的买方压力信号
- 趋势状态分类(从 analyze_market_trend 方法可以看到):
1
2
3
4
5
6
7
8
9
10
| if ratio > 1.5:
trend = "Strong Bullish" # 强烈看涨(买方压力很大)
elif ratio > 1.2:
trend = "Bullish" # 看涨(买方压力较大)
elif ratio < 0.67: # 1/1.5
trend = "Strong Bearish" # 强烈看跌(卖方压力很大)
elif ratio < 0.83: # 1/1.2
trend = "Bearish" # 看跌(卖方压力较大)
else:
trend = "Neutral" # 中性(买卖压力平衡)
|
- 深度分析参数(Depth Analysis)显示三个价格区间的买卖情况:
1
2
3
4
5
| price_ranges = {
'near': 0.001, # 当前价格±0.1%的区间
'mid': 0.005, # 当前价格±0.5%的区间
'far': 0.01 # 当前价格±1%的区间
}
|
每个区间的状态分类:
1
2
3
4
5
6
7
8
9
10
| if ratio > 1.5:
status = 'Strong Buy' # 强烈买入信号
elif ratio > 1.2:
status = 'Buy' # 买入信号
elif ratio < 0.67:
status = 'Strong Sell' # 强烈卖出信号
elif ratio < 0.83:
status = 'Sell' # 卖出信号
else:
status = 'Neutral' # 中性
|
特殊状态:
- No Orders: 该区间没有订单
- Buy Only: 只有买单没有卖单
- Sell Only: 只有卖单没有买单
- Error: 计算出错
所以在你的例子中,ETHUSDT 显示 “Strong Bullish B/A Ratio: 6.88” 表示:
- 市场上买单量是卖单量的6.88倍
- 这表明有很强的买方压力
- 可能预示着价格上涨的趋势
需要我详细解释某个具体参数吗?
下面代码 改为 如果打开页面时的数据 如果小于最高值。则显示跌,且计算跌幅率。 如果这个值是最高的。则显示涨。且计算涨幅率。
1
2
3
4
5
6
7
8
9
10
11
12
13
14
15
16
17
18
19
20
21
22
23
24
25
26
27
28
29
30
31
32
33
34
35
36
37
38
39
40
41
| 【 let ethStatsText = '', btcStatsText = '', solStatsText = '', xauStatsText = '';
if (ethData) {
const ethPrices = ethData.prices.map((price, index) => ({
x: ethData.times[index],
y: price
}));
const ethHighestPrice = Math.max(...ethData.prices);
const ethLowestPrice = Math.min(...ethData.prices);
const ethPercentageChange = ((ethData.prices[ethData.prices.length - 1] - ethData.prices[0]) / ethData.prices[0] * 100).toFixed(2);
ethStatsText = `ETH - 最高值: ${ethHighestPrice} USD, 最低值: ${ethLowestPrice} USD, 涨幅: ${ethPercentageChange}%`;
ethDatasets.push({
label: 'ETH Price',
backgroundColor: 'rgba(255, 99, 132, 0.2)',
borderColor: 'rgb(255, 0, 207)',
data: ethPrices,
fill: false,
borderWidth: 1
});
}
if (btcData) {
const btcPrices = btcData.prices.map((price, index) => ({
x: btcData.times[index],
y: price
}));
const btcHighestPrice = Math.max(...btcData.prices);
const btcLowestPrice = Math.min(...btcData.prices);
const btcPercentageChange = ((btcData.prices[btcData.prices.length - 1] - btcData.prices[0]) / btcData.prices[0] * 100).toFixed(2);
btcStatsText = `BTC - 最高值: ${btcHighestPrice} USD, 最低值: ${btcLowestPrice} USD, 涨幅: ${btcPercentageChange}%`;
btcDatasets.push({
label: 'BTC Price',
backgroundColor: 'rgba(255, 182, 193, 0.2)',
borderColor: 'rgb(255, 105, 180)',
data: btcPrices,
fill: false,
borderWidth: 1
});
}】
|
↑↓
在下面prices webserver中添加实现数据存储的功能。不改变原有服务, 实现在不同设备和浏览器间同步数据
1
2
3
4
5
6
7
8
9
10
11
12
13
14
15
16
17
| from flask import Flask, request, jsonify
from datetime import datetime, timedelta
import re
app = Flask(__name__)
#nohup python price_web.py > price_web.out 2>&1 & --> location /prices {proxy_pass http://127.0.0.1:5000 --> https://2urs.com/trading/eth.html
def fetch_prices(log_file, keyword, duration):
......
@app.route('/prices/<crypto>')
def prices(crypto):
......
if __name__ == '__main__':
app.run(debug=True)
|
基本可以跑了,在原来的基础上改下,html 收藏记录 格式改为 【行头:价格 跌6% 涨7% 涨9% 时间
3830 xx 4098 xx 2024/12/12 22:51:52】 顺便的改下相应的前 后端代码
后端报错了 你删掉之前的 表 按需求重新建表 然后 @app.route(’/prices/calculations’, methods=[‘POST’]) @app.route(’/prices/calculations’, methods=[‘GET’]) 吧
基本可以跑了,在原来的基础上改下,html 收藏记录 格式改为
1
2
3
| 【行头:价格 跌6% 涨7% 涨9% 时间 备注
3830 xx 4098 xx 2024/12/12 22:51:52 xxx 】
|
备注默认空,在点收藏时,如有写备注。则增加备注。 顺便的改下相应的前 后端代码
基本可以跑了,在原来的基础上改下,html 收藏记录 格式改为 【行头:价格 跌6% 涨7% 涨9% 时间 备注
3830 xx 4098 xx 2024/12/12 22:51:52 xxx 】,备注默认空,在点收藏时,如有写备注。则增加备注。 顺便的改下相应的前 后端代码 。
1
2
3
4
5
6
7
8
9
10
11
12
13
14
15
16
17
18
19
20
21
22
23
24
25
26
27
28
29
30
31
32
33
34
35
36
37
38
39
40
41
42
43
44
45
46
47
48
49
50
51
52
53
54
55
56
57
58
59
60
61
62
63
64
65
66
67
68
69
70
71
72
73
74
75
76
77
78
79
80
81
82
83
84
85
86
87
88
89
90
91
92
93
94
95
96
97
98
99
100
101
102
103
104
105
106
107
108
109
110
111
112
113
114
115
116
117
118
119
120
121
122
123
124
125
126
127
128
129
130
131
132
133
134
135
136
137
138
139
140
141
142
143
144
145
146
147
148
149
150
151
152
153
154
155
156
157
158
159
160
| html代码:
/* 计算器*/
.container {
margin: 20px;
font-family: Arial, sans-serif;
}
.result {
margin-top: 10px;
font-size: 16px;
}
.favorites {
margin-top: 20px;
border: 1px solid #ccc;
padding: 10px;
max-height: 500px;
overflow-y: auto;
}
.favorite-item {
background-color: #f8f8f8;
}
.favorite-item:hover {
background-color: #f0f0f0;
}
.error-message {
color: red;
margin-top: 10px;
}
</style>
....
<!-- 计算器 -->
<div class="container">
<input type="number" id="inputNumber" placeholder="请输入数字">
<button onclick="calculate()">计算</button>
<button onclick="saveFavorite()">收藏</button>
<div class="result" id="result7"></div>
<div class="favorites">
<div id="favoritesList"></div>
</div>
<div id="errorMessage" class="error-message"></div>
</div>
<script>
// 计算器
let currentCalculation = {
input: 0,
drop6: 0,
result7: 0,
result9: 0
};
// 页面加载时获取已保存的收藏
window.onload = function() {
loadFavorites();
};
async function loadFavorites() {
try {
const response = await fetch('/prices/calculations');
if (!response.ok) {
throw new Error('获取数据失败');
}
const calculations = await response.json();
displayFavorites(calculations);
} catch (error) {
showError('加载收藏记录失败: ' + error.message);
}
}
function calculate() {
const input = document.getElementById('inputNumber').value;
const number = parseInt(input);
if (!isNaN(number)) {
const drop6 = Math.round(number * (1 - 0.06)); // 跌6%
const result7 = Math.round(number * (1 + 0.07)); // 涨7%
const result9 = Math.round(number * (1 + 0.09)); // 涨9%
document.getElementById('result7').innerHTML =
`跌6%:${drop6} | 涨7%:${result7} | 涨9%:${result9}`;
currentCalculation = {
input: number,
drop6: drop6,
result7: result7,
result9: result9
};
} else {
showError('请输入有效的数字!');
}
}
async function saveFavorite() {
if (currentCalculation.input === 0) {
showError('请先进行计算!');
return;
}
try {
const response = await fetch('/prices/calculations', {
method: 'POST',
headers: {
'Content-Type': 'application/json',
},
body: JSON.stringify(currentCalculation)
});
if (!response.ok) {
throw new Error('保存失败');
}
await loadFavorites(); // 重新加载收藏列表
showError('保存成功!', false);
} catch (error) {
showError('保存失败: ' + error.message);
}
}
function displayFavorites(calculations) {
const favoritesList = document.getElementById('favoritesList');
// 创建表格样式的布局
let html = `
<div class="favorite-header" style="display: grid; grid-template-columns: repeat(5, 1fr); font-weight: bold; background-color: #f0f0f0; padding: 8px; margin-bottom: 10px;">
<div>价格</div>
<div>跌6%</div>
<div>涨7%</div>
<div>涨9%</div>
<div>时间</div>
</div>
`;
// 添加数据行
html += calculations.map(item => `
<div class="favorite-item" style="display: grid; grid-template-columns: repeat(5, 1fr); padding: 8px; border-bottom: 1px solid #eee;">
<div>${item.input}</div>
<div>${item.drop6}</div>
<div>${item.result7}</div>
<div>${item.result9}</div>
<div>${item.timestamp}</div>
</div>
`).join('');
favoritesList.innerHTML = html;
}
function showError(message, isError = true) {
const errorDiv = document.getElementById('errorMessage');
errorDiv.style.color = isError ? 'red' : 'green';
errorDiv.textContent = message;
setTimeout(() => {
errorDiv.textContent = '';
}, 3000);
}
</script>
|
后端代码【
1
2
3
4
5
6
7
8
9
10
11
12
13
14
15
16
17
18
19
20
21
22
23
24
25
26
27
28
29
30
31
32
33
34
35
36
37
38
39
40
41
42
43
44
45
46
47
48
49
50
51
52
53
54
55
56
57
58
59
60
61
62
63
64
65
66
67
68
69
70
71
72
73
74
75
76
77
78
79
80
81
82
83
84
85
86
| from flask import Flask, request, jsonify
from datetime import datetime, timedelta
import re
import sqlite3
app = Flask(__name__)
......
# 初始化数据库,删除旧表并创建新表
def init_db():
conn = sqlite3.connect('calculations.db')
c = conn.cursor()
# 删除旧表(如果存在)
#c.execute('DROP TABLE IF EXISTS calculations')
# 创建新表
c.execute('''
CREATE TABLE calculations
(id INTEGER PRIMARY KEY AUTOINCREMENT,
input_value INTEGER,
drop_6 INTEGER,
result_7 INTEGER,
result_9 INTEGER,
timestamp DATETIME DEFAULT CURRENT_TIMESTAMP)
''')
conn.commit()
conn.close()
# 新增的计算记录相关路由
# 保存计算结果
@app.route('/prices/calculations', methods=['POST'])
def save_calculation():
try:
data = request.get_json()
input_value = data.get('input')
drop_6 = data.get('drop6')
result_7 = data.get('result7')
result_9 = data.get('result9')
conn = sqlite3.connect('calculations.db')
c = conn.cursor()
c.execute('''
INSERT INTO calculations (input_value, drop_6, result_7, result_9)
VALUES (?, ?, ?, ?)
''', (input_value, drop_6, result_7, result_9))
conn.commit()
conn.close()
return jsonify({'status': 'success', 'message': 'Calculation saved'})
except Exception as e:
return jsonify({'status': 'error', 'message': str(e)}), 500
# 获取所有计算记录
@app.route('/prices/calculations', methods=['GET'])
def get_calculations():
try:
conn = sqlite3.connect('calculations.db')
c = conn.cursor()
c.execute('''
SELECT input_value, drop_6, result_7, result_9,
strftime('%Y/%m/%d %H:%M:%S', timestamp, 'localtime') as timestamp
FROM calculations
ORDER BY timestamp DESC
''')
rows = c.fetchall()
conn.close()
calculations = []
for row in rows:
calculations.append({
'input': row[0],
'drop6': row[1],
'result7': row[2],
'result9': row[3],
'timestamp': row[4]
})
return jsonify(calculations)
except Exception as e:
return jsonify({'status': 'error', 'message': str(e)}), 500
if __name__ == '__main__':
#if not os.path.exists('calculations.db'):
# init_db()
app.run(debug=True)
】
|
怎么快速的修改行记录 ,删除行
html 输出显示
价格 跌6% 涨7% 涨9% 备注 记录时间
3750 3525 4013 4088 12-12 10:45 大单进入 2024/12/13 09:17:12
1
2
3
4
5
6
7
8
9
10
11
12
13
14
15
16
17
18
19
20
21
22
23
24
25
26
27
28
29
30
31
32
33
| # 初始化数据库,删除旧表并创建新表
def init_db():
conn = sqlite3.connect('calculations.db')
c = conn.cursor()
# 删除旧表(如果存在)
c.execute('DROP TABLE IF EXISTS calculations')
# 创建新表,增加 remark 字段
c.execute('''
CREATE TABLE calculations
(id INTEGER PRIMARY KEY AUTOINCREMENT,
input_value INTEGER,
drop_6 INTEGER,
result_7 INTEGER,
result_9 INTEGER,
remark TEXT,
timestamp DATETIME DEFAULT CURRENT_TIMESTAMP)
''')
conn.commit()
conn.close()
...
if __name__ == '__main__':
init_db()
app.run(debug=True)
3750
3525
4013
4088
12-11 22:45 大单进入
|
点计算 收藏按钮功能ok,但点删除记录报错
DELETE https://test.com/prices/calculations/undefined 404 (Not Found)
后端代码
1
2
3
4
5
6
7
8
9
10
11
12
13
14
15
16
17
18
19
20
21
22
23
24
25
26
27
28
29
30
31
32
33
34
35
36
37
38
39
40
41
42
| 【
......
#修改记录接口 提供一个 PUT 方法,通过记录的 id 来更新某条记录的内容
@app.route('/prices/calculations/<int:record_id>', methods=['PUT'])
def update_calculation(record_id):
"""更新指定记录"""
try:
data = request.get_json()
input_value = data.get('input_value')
drop_6 = data.get('drop_6')
result_7 = data.get('result_7')
result_9 = data.get('result_9')
remark = data.get('remark', '')
conn = sqlite3.connect('calculations.db')
c = conn.cursor()
c.execute('''
UPDATE calculations
SET input_value = ?, drop_6 = ?, result_7 = ?, result_9 = ?, remark = ?
WHERE id = ?
''', (input_value, drop_6, result_7, result_9, remark, record_id))
conn.commit()
conn.close()
return jsonify({'status': 'success', 'message': 'Record updated successfully'})
except Exception as e:
return jsonify({'status': 'error', 'message': str(e)}), 500
# 删除记录接口 提供一个 DELETE 方法,通过记录的 id 来删除某条记录
@app.route('/prices/calculations/<int:record_id>', methods=['DELETE'])
def delete_calculation(record_id):
"""删除指定记录"""
try:
conn = sqlite3.connect('calculations.db')
c = conn.cursor()
c.execute('DELETE FROM calculations WHERE id = ?', (record_id,))
conn.commit()
conn.close()
return jsonify({'status': 'success', 'message': 'Record deleted successfully'})
except Exception as e:
return jsonify({'status': 'error', 'message': str(e)}), 500】
|
前端代码 【
1
2
3
4
5
6
7
8
9
10
11
12
13
14
15
16
17
18
19
20
21
22
23
24
25
26
27
28
29
30
31
32
33
34
35
36
37
38
39
40
41
42
43
44
45
46
47
48
49
50
51
52
53
54
55
56
57
58
59
60
61
62
63
64
65
66
67
68
69
70
71
72
73
74
75
76
77
78
79
80
81
82
83
84
85
86
87
88
89
90
91
92
93
94
95
96
97
98
99
100
101
102
103
104
105
106
107
108
109
110
111
112
113
114
115
116
117
118
119
120
121
122
123
124
125
126
127
128
129
130
131
132
133
134
135
136
137
138
139
140
141
142
143
144
145
146
147
148
149
150
151
152
153
154
155
156
157
158
159
160
161
162
163
164
165
166
167
168
169
170
171
172
173
174
| ......
<!-- 计算器 -->
<div class="container">
<input type="number" id="inputNumber" placeholder="请输入数字">
<button onclick="calculate()">计算</button>
<input type="text" id="remark" placeholder="备注 (可选)">
<button onclick="saveFavorite()">收藏</button>
<div class="result" id="result7"></div>
<div class="favorites">
<div id="favoritesList"></div>
</div>
<div id="errorMessage" class="error-message"></div>
</div>
......
<script>
......
// 保存当前计算的信息
let currentCalculation = {
input: 0,
drop6: 0,
result7: 0,
result9: 0,
remark: '' // 默认备注为空
};
// 页面加载时获取已保存的收藏
window.onload = function () {
loadFavorites();
};
// 删除功能
async function deleteRecord(id) {
if (!confirm("确定要删除这条记录吗?")) {
return;
}
try {
const response = await fetch(`/prices/calculations/${id}`, {
method: 'DELETE'
});
if (!response.ok) {
throw new Error('删除失败');
}
await loadFavorites(); // 重新加载收藏列表
alert('记录删除成功!');
} catch (error) {
alert('删除失败: ' + error.message);
}
}
// 加载收藏记录
async function loadFavorites() {
try {
const response = await fetch('/prices/calculations');
if (!response.ok) {
throw new Error('获取数据失败');
}
const calculations = await response.json();
displayFavorites(calculations);
} catch (error) {
alert('加载收藏记录失败: ' + error.message);
}
}
// 计算逻辑
function calculate() {
const input = document.getElementById('inputNumber').value;
const number = parseInt(input);
if (!isNaN(number)) {
const drop6 = Math.round(number * (1 - 0.06)); // 跌6%
const result7 = Math.round(number * (1 + 0.07)); // 涨7%
const result9 = Math.round(number * (1 + 0.09)); // 涨9%
document.getElementById('result7').innerHTML =
`跌6%:${drop6} | 涨7%:${result7} | 涨9%:${result9}`;
currentCalculation = {
input: number,
drop6: drop6,
result7: result7,
result9: result9,
remark: '' // 默认备注为空
};
} else {
showError('请输入有效的数字!');
}
}
// 保存收藏记录
async function saveFavorite() {
if (currentCalculation.input === 0) {
showError('请先进行计算!');
return;
}
const remarkInput = document.getElementById('remark').value.trim();
currentCalculation.remark = remarkInput || ''; // 保存备注
try {
const response = await fetch('/prices/calculations', {
method: 'POST',
headers: {
'Content-Type': 'application/json',
},
body: JSON.stringify(currentCalculation)
});
if (!response.ok) {
throw new Error('保存失败');
}
await loadFavorites(); // 重新加载收藏列表
showError('保存成功!', false);
} catch (error) {
showError('保存失败: ' + error.message);
}
}
// 显示收藏记录
function displayFavorites(calculations) {
const favoritesList = document.getElementById('favoritesList');
let html = `
<div class="favorite-header" style="display: grid; grid-template-columns: repeat(7, 1fr); font-weight: bold; background-color: #f0f0f0; padding: 8px; margin-bottom: 10px;">
<div>价格</div>
<div>跌6%</div>
<div>涨7%</div>
<div>涨9%</div>
<div>备注</div>
<div>记录时间</div>
<div>操作</div>
</div>
`;
html += calculations.map(item => `
<div class="favorite-item" style="display: grid; grid-template-columns: repeat(7, 1fr); padding: 8px; border-bottom: 1px solid #eee;">
<div>${item.input}</div>
<div>${item.drop6}</div>
<div>${item.result7}</div>
<div>${item.result9}</div>
<div>${item.remark || ''}</div>
<div style="color: #D3D3D3;">${item.timestamp}</div>
<div>
<button onclick="editRecord(${item.id})">编辑</button>
<button onclick="deleteRecord(${item.id})">删除</button>
</div>
</div>
`).join('');
favoritesList.innerHTML = html;
}
// 显示错误或成功消息
function showError(message, isError = true) {
const errorDiv = document.getElementById('errorMessage');
errorDiv.style.color = isError ? 'red' : 'green';
errorDiv.textContent = message;
setTimeout(() => {
errorDiv.textContent = '';
}, 3000);
}
</script>】
|
1
2
3
4
5
6
7
8
9
10
11
12
13
14
15
16
17
18
19
20
21
22
23
24
25
26
27
28
29
30
31
32
33
34
35
36
37
38
39
40
41
42
43
44
45
46
47
48
49
50
51
52
53
54
55
56
57
58
59
60
61
62
63
64
65
66
| from binance.client import Client
import pandas as pd
import concurrent.futures
api_key = '5nV9ChLDDCSg 1gvvRCAaF5L9gZtRx20RM8CMxQ8'
api_secret = '5xHbdWenlYBF8 VoZe7tyxBMTZmblIrrO0K'
client = Client(api_key, api_secret)
def get_large_orders(symbol, threshold):
"""
获取指定交易对的大单买入和卖出订单。
:param symbol: 交易对符号,例如'BTCUSDT'或'ETHUSDT'
:param threshold: 定义大单的数量阈值
:return: 包含大单买入和卖出订单的DataFrame
"""
try:
order_book = client.get_order_book(symbol=symbol, limit=5000)
large_bids = [(bid[0], float(bid[1])) for bid in order_book['bids'] if float(bid[1]) > threshold]
large_asks = [(ask[0], float(ask[1])) for ask in order_book['asks'] if float(ask[1]) > threshold]
# 创建DataFrame
bids_df = pd.DataFrame(large_bids, columns=['Price', 'Quantity']).assign(Type='Bid', Symbol=symbol)
asks_df = pd.DataFrame(large_asks, columns=['Price', 'Quantity']).assign(Type='Ask', Symbol=symbol)
return pd.concat([bids_df, asks_df])
except Exception as e:
print(f"Error fetching order book for {symbol}: {e}")
return pd.DataFrame()
def fetch_orders_for_symbols(symbols_thresholds):
"""
并行获取多个交易对的大单订单信息。
:param symbols_thresholds: 交易对与其对应的大单阈值的字典
:return: 包含所有大单信息的DataFrame
"""
with concurrent.futures.ThreadPoolExecutor() as executor:
future_to_symbol = {executor.submit(get_large_orders, symbol, threshold): symbol for symbol, threshold in
symbols_thresholds.items()}
results = []
for future in concurrent.futures.as_completed(future_to_symbol):
symbol = future_to_symbol[future]
try:
data = future.result()
results.append(data)
except Exception as e:
print(f"Error processing {symbol}: {e}")
return pd.concat(results, ignore_index=True)
# 指定要查询的交易对和各自的阈值
symbols_thresholds = {
'BTCUSDT': 10, # BTC的大单阈值
'ETHUSDT': 1000 # ETH的大单阈值
}
# 获取并处理订单数据
large_orders_df = fetch_orders_for_symbols(symbols_thresholds)
# 打印结果
if not large_orders_df.empty:
print(large_orders_df.to_string(index=False))
else:
print("没有找到符合条件的大单。")
|
能优化下吗 ? 比如按最近1天的数据,出现好几次v v v这样的? 找出现在的eth波段 ,【每个v的 最低 最高 幅度是多少%?时间区间是多少 比如1小时。从2024-12-14 05:21:11 -2024-12-14 11:21:11 这种格式 ;建议买价 卖价 盈利多少 $? 】可用 binance.client
你是说REST API 方法 最多只能取1000条订单量 ?有更好的方式 获取近1天时间段内eth 和btc的已经成交的大单数据。
– 自买 找大户 跟随
https://x.com/ai_9684xtpa
https://intel.arkm.com/explorer/address/0x148990D87C467Ab1E9fF3435D7E4Ac0909e640c8
– 网格
– 跟单
现货
https://www.binance.com/zh-CN/copy-trading/lead-details/4291250816309271552?timeRange=7D
合约
https://www.binance.com/zh-CN/copy-trading/lead-details/3725468878881937408?timeRange=7D
https://www.binance.com/zh-CN/copy-trading/lead-details/3737737591787196160?timeRange=7D
创建一个chrome浏览器插件项目,它的作用是: 获取当前打开页面的视频或音频文件,(有过滤器: 大于100 KB,小于此值的视频将不会被捕获)
点插件图标 , 显示当前页面的文件的过滤的mp4或mp3媒体文件 【嗅探到当前页面的文件的过滤的mp4或者mp3等媒体文件,】 显示信息窗口【窗口大小 宽度从浏览器当前插件图标位置开始,向左608Px,每个文件的信息高度60px】 ,每个文件的信息为:播放按钮 文件名 下载按钮 复制按钮 ,
下面是具体描述:
播放按钮: 点击可以播放下窗口视频
文件名:mp4unnamed-file
下载按钮(按钮上显示文件大小,点击下载弹出到下载页面【后台服务页面,比如当前ip是中国 test.com/cn/staticdownloader,加拿大 则test.com/ca/staticdownloader 支持日本多国页面
1
2
3
4
5
6
7
8
9
10
| IN hi;
US en;
ID id;
BR pt;
PK ur;
NG en;
BD bn;
RU ru;
JP ja;
ES es;】。;
|
下载页面有3个区块:1 Chrome Web Store 、 Edge Add-on按钮分别链接到https://chromewebstore.google.com/detail/插件 和https://microsoftedge.microsoft.com/addons/detail/插件 主页面;2: 静态视频下载器区域 保存按钮。 点保存则下载视频到本地 并有圆圈下载进度,可以指定 3个挡位的下载并发请求 比如点 1 2 3 档,默认选择2挡位。3 FAQ 区域, 一些问答 】),
复制按钮(复制文件连接)。
1
2
3
4
5
6
7
8
9
10
11
12
13
14
15
16
17
18
19
20
21
22
23
24
25
26
27
28
29
30
31
32
33
34
35
36
37
38
39
40
41
42
43
44
45
46
47
48
49
50
51
52
53
54
55
56
57
| 【 def get_large_orders(symbol, threshold):
#获取指定交易对的大单买入和卖出订单。
#:param symbol: 交易对符号,例如'BTCUSDT'或'ETHUSDT'
#:param threshold: 定义大单的数量阈值
#:return: 包含大单买入和卖出订单的DataFrame
try:
order_book = client.get_order_book(symbol=symbol, limit=5000)
large_bids = [(bid[0], float(bid[1])) for bid in order_book['bids'] if float(bid[1]) > threshold]
large_asks = [(ask[0], float(ask[1])) for ask in order_book['asks'] if float(ask[1]) > threshold]
# 创建DataFrame
bids_df = pd.DataFrame(large_bids, columns=['Price', 'Quantity']).assign(Type='Bid', Symbol=symbol)
asks_df = pd.DataFrame(large_asks, columns=['Price', 'Quantity']).assign(Type='Ask', Symbol=symbol)
return pd.concat([bids_df, asks_df])
except Exception as e:
print(f"Error fetching order book for {symbol}: {e}")
return pd.DataFrame()
def fetch_orders_for_symbols(symbols_thresholds):
#并行获取多个交易对的大单订单信息。
#:param symbols_thresholds: 交易对与其对应的大单阈值的字典
#:return: 包含所有大单信息的DataFrame
with concurrent.futures.ThreadPoolExecutor() as executor:
future_to_symbol = {executor.submit(get_large_orders, symbol, threshold): symbol for symbol, threshold in
symbols_thresholds.items()}
results = []
for future in concurrent.futures.as_completed(future_to_symbol):
symbol = future_to_symbol[future]
try:
data = future.result()
results.append(data)
except Exception as e:
print(f"Error processing {symbol}: {e}")
return pd.concat(results, ignore_index=True)
# 指定要查询的交易对和各自的阈值
symbols_thresholds = {
'BTCUSDT': 10, # BTC的大单阈值
'ETHUSDT': 200 # ETH的大单阈值
}
# 获取并处理订单数据
large_orders_df = fetch_orders_for_symbols(symbols_thresholds)
# 打印结果
if not large_orders_df.empty:
print(large_orders_df.to_string(index=False))
else:
print("没有找到符合条件的大单。")】
|
优化下代码 ,每1分钟查询一次, 查出下面类似数据的基础上,在加上当前北京具体时间,当前实盘价位,
1
2
3
4
5
6
| 【 Price Quantity Type Symbol
104000.00000000 25.27583 Bid BTCUSDT
105000.00000000 22.93544 Ask BTCUSDT
3860.00000000 241.12840 Bid ETHUSDT
3985.00000000 218.70760 Ask ETHUSDT
T】
|
把它存入 sqlite3 ,在前端展示图出来 体现,要体现某时刻,买盘 与 卖盘的哪个强( 比如 2024-12-16 22:30 时刻,买盘 大于 卖盘 绿色块大于红色块 。或者你熟悉的通用方式来展现)
买盘 与 卖盘的哪个强
现在没有btc eth 价格曲线数据, 你能把@pricev2.html btc和eth的图表 按上下并列的方式,放在 Crypto Order Book Analysis 图表区域的右边,也就是 Update Interval:的下面。现在 是空白的。怎样把 pricev2.html 的btc和 eth2个价格曲线图 放在这个空白区域呢? 最好统一放在display.py 里面。这样整个画面空间得到利用。
https://2urs.com/prices/btc?duration=?
1: 现在价格你是模拟的数据,用下面的数据:
https://test.com/prices/btc?duration=?
https://test.com/prices/eth?duration=?
?为多少个小时,可以选择的参数: 2 4 8 12 16 20 24 48 72 96 720 1440
2:Price Charts 这个图标区域可以网上一点点,跟Crypto Order Book Analysis 图表区域的顶端齐平 这样好看点,
3: Update Interval:的这个选择框宽度可以少点 建议200px , 然后把btc eth价格图标的时间选择框 放在Update Interval:的这个选择框的右边 , 水平齐平。
4: Crypto Order Book Analysis 图表、Price Charts 图表、BTCUSDT Large Orders (>10 BTC) 、ETHUSDT Large Orders (>500 ETH) 要做到同时刷新
1:Price Charts 这个图表区域 跟Crypto Order Book Analysis 宽度 一样 而且是水平齐平显示(Crypto Order Book Analysis在左 Price Charts在右) 。 具体宽度跟BTCUSDT Large Orders (>10 BTC) 、ETHUSDT Large Orders (>500 ETH) 的宽度、做到统一。
2:Crypto Order Book Analysis 的btc的volume 为什么显示为0.210的15次方、0.410的15 等。 eth的volume 为什么显示为0.510的16次方 、110的16 等。这个值具体是指什么,就要显示对。
3:Crypto Order Book Analysis 的 Time和年月时分秒可以放一行显示 这样可以减少占用高度空间。BTCUSDT asks BTCUSDT bids ETHUSDT asks ETHUSDT bids 改为放在图表的最下面(时间的下面),水平齐平放置。
1:Price Charts 这个图表区域 跟Crypto Order Book Analysis 宽度、高度一样 而且是水平齐平显示(Crypto Order Book Analysis在左 Price Charts在右)现在Price Charts的高度低了点 。 具体宽度跟BTCUSDT Large Orders (>10 BTC) 、ETHUSDT Large Orders (>500 ETH) 的宽度、做到统一。
2 Crypto Order Book Analysis 的btc的volume 为什么显示为10P 20P等。 eth的volume 为什么显示为20P 40P 等。这个值具体是指什么,就要显示对。 之前的那2个参数为什么没有了?
1:买卖盘强度表中 btc 和eth的y volume 显示很大 500,000,000,000,000,000 类似这样的。 这个volume 具体是指什么,写明白是什么volume 。 然后数值是否正确,如果数值大请用bian的标准表示。
其它功能不要动了。
代码有点小缺陷,cursor 改了3 版本,还不如第原来的, 怎么回滚代码到第一版呢
1:买卖盘强度表中 btc 和eth的y volume 显示很大 500,000,000,000,000,000 类似这样的。 这个volume 具体是指什么,写明白是什么volume 。 然后数值是否正确,如果数值大请用bi an的标准表示。2 买卖盘强度表中 btc 和eth的图表下面时间 年月时分秒可以放一行显示 2024 12/17 时分秒 这样可以减少占用高度空间
其它功能不要动了。
第一问题: 数据库存在多条重复的值,
1
2
3
4
5
6
7
| Time Type Price Quantity
2024-12-17 18:19:03 Ask 108000.00 127.59
2024-12-17 18:19:01 Ask 108000.00 127.59
2024-12-17 18:18:31 Ask 108000.00 127.59
2024-12-17 18:18:30 Ask 108000.00 127.59
2024-12-17 18:18:00 Ask 108000.00 127.59
2024-12-17 18:17:58 Ask 108000.00 127.59
|
比如30秒去获取ask bid 单子。 但一直没有成交。 每次查询 就会有一样的数据,这样存入数据库。
要体现现在买盘 与 卖盘的哪个强 ,会不会对现在的计算逻辑有影响。
现在代码是 计算买卖盘强度 考虑因素:1. 订单量大小 2. 价格偏离度 3. 订单集中度 4. 时间衰减。 怎样更加准确的计算买卖盘强度呢。
第2个问题:如果单子 Quantity有大于100btc,和 1000的eth 单子。要考虑发邮件。
1
2
3
4
5
6
7
8
9
10
11
12
13
14
15
16
17
18
| # 电子邮件发送函数
def send_email7(subject, content):
sender = 'htmonitor1@126.com'
receiver = 'hnkady@126.com'
password = 'xxxxxx'
message = MIMEMultipart()
message['From'] = sender
message['To'] = receiver
message['Subject'] = subject
message.attach(MIMEText(content, 'plain', 'utf-8'))
server = smtplib.SMTP('smtp.126.com', 25) # SMTP 服务器地址和端口号
server.starttls()
server.login(sender, password)
text = message.as_string()
server.sendmail(sender, receiver, text)
server.quit()
|
第2个问题: 同时再前端要做个闪烁报警的功能。直达大单消失
第4个问题:
1
2
3
4
5
6
7
8
9
10
11
| symbols_thresholds = {
'BTCUSDT': 10,
'ETHUSDT': 200
}```
这个阈值,会改动,如果改动会影响你的计算逻辑吗。 如果不影响,如何只改一个地方,做到前后端显示一样的数据。(前端有过滤数据和BTCUSDT Large Orders (>10 BTC) 、ETHUSDT Large Orders (>500 ETH) 显示呢 )
----
第1个问题:
(前端图标标题BTCUSDT Large Orders (>10 BTC) 、ETHUSDT Large Orders (>500 ETH)
配置
|
THRESHOLDS = {
‘BTCUSDT’: {
‘display’: 10, # 显示阈值
‘alert’: 100, # 警报阈值
‘fetch’: 5 # 获取数据阈值
}, )
```
然后你前端页面展示单子有
1
2
3
4
5
6
7
8
9
10
11
12
13
14
15
16
17
18
19
20
21
22
23
24
| Time Type Price Quantity
2024-12-17 18:47:28 Bid 106888.00 5.05
你这个不统一吧? 要改为统一的。 比如 'fetch': 5 ```。
抓取大于5的单子,前端显示'display': 5, 那也要把抓取大于5的单子展示出来,如果抓取大于10的单子,前端显示'display': 10, 那也要把抓取大于10的单子展示出来,
eth也一样。
注意最重要的是为 计算买卖盘强度准确度 服务。就是这个系统要做到要体现当下,买盘 与 卖盘的哪个强 这个要准确。
第2个问题:
比如打开页面,btc 买卖强度图下面有个 2024 12/17 11:01:30 这个时间基本不用动 ,你把这个时间改为北京时间就行。
第3个问题:写个测试脚本,插入数据库指定id的模拟数据,来测试邮件报警 和前端闪烁报警功能。 和需要删除指定数据功能。
btc和eth的 text=f"{trend}\nB/A Ratio: {ratio:.2f}", 和depth_text = "Depth Analysis:\n" + "\n".join 相关信息直接在柱子图上,有点乱。
先说btc的 ,把B/A Ratio 和Depth Analysis 放在这个买卖强度图的 BTCUSDT的下面,这样规整点。btc买卖强度图其它不用变。
样注意最重要的是为 计算买卖盘强度准确度 服务。就是这个系统要做到要体现当下,买盘 与 卖盘的哪个强 这个要准确。
eth的也一样改下。注意btc和eth的买卖强度图是分开的。
--
入测试订单 (ID: 18781): ETHUSDT Bid 400 @ 3000
获取测试数据时发生错误: ```Execution failed on sql '
SELECT * FROM large_orders
WHERE id IN (18778,18779,18780,18781)
ORDER BY timestamp DESC
': no such column: id
|
当前测试数据:
Empty DataFrame
Columns: []
Index: []
测试过程中发生错误: ‘symbol’
删除测试数据时发生错误: no such column: id 需要在原库的表插入测试数据,并记住id 。支持删除新增的测试数据。
直接改了数据库,现在网页前端:
btc 有比 2024-12-17 20:33:38 Bid 106969.99 108.53
eth 有比2024-12-17 20:33:09 Bid 3950.00 1517.03
为什么没有邮件报警【邮箱密码是改过对的了】和前端闪烁报警
1
2
3
4
5
6
7
8
9
10
11
12
13
14
15
16
17
18
| Time: 2024-12-17 12:50:01
邮件发送失败: (554, b'IP is rejected, smtp auth error limit exceed,126 gzga-smtp-mtada-g1-4,_____wDXf6_lcWFnmg6uAQ--.63551S0 1734439397')
INFO:__main__:发送大单报警邮件: Large Ask Order Detected!
Symbol: ETHUSDT
Price: 4100.0
Quantity: 1340.7486
Time: 2024-12-17 12:49:17
Saved 9 records to database
Saved 7 records to database
ERROR:__main__:更新数据时发生错误: module 'dash.html' has no attribute 'Style'
ERROR:__main__:Traceback (most recent call last):
File "E:\act_now\IncrementalSteps\bid_ask\main.py", line 78, in update_all_data
btc_table = display.create_large_orders_table(df, 'BTCUSDT', 10, current_alerts)
^^^^^^^^^^^^^^^^^^^^^^^^^^^^^^^^^^^^^^^^^^^^^^^^^^^^^^^^^^^^^^^^^^^^
File "E:\act_now\IncrementalSteps\bid_ask\bid_ask\display.py", line 200, in create_large_orders_table
html.Style(app_css),
^^^^^^^^^^
AttributeError: module 'dash.html' has no attribute 'Style'
|
优化下:1 邮件报警,每1个大单只邮件1次报警(一模一样的数据不用在邮件报警),不能重复发。 可以给表加字段。设置是否邮件警告。
2 html 报错了改下
1
2
3
4
5
6
7
8
9
10
11
12
13
14
15
| 邮件发送失败: (554, b'IP is rejected, smtp auth error limit exceed,126 gzga-smtp-mtada-g0-1,_____wDnz+NrdGFncJk_Ag--.37529S0 1734440043')
INFO:__main__:发送大单报警邮件: Large Ask Order Detected!
Symbol: ETHUSDT
Price: 4100.0
Quantity: 1531.6208
Time: 2024-12-17 17:09:29 。```
你这个是运行程序,把库中所有
符合的记录都发邮件,肯定不对啊,不是当时的不要发。是要当时 比如前端页面现在北京时间20:59 是在这个10分钟内的 做符合阈值判断。
超过阈值的,邮件报警,每1个大单只邮件1次报警(一模一样的数据不用在邮件报警),不能重复发。
html 报警的逻辑也是一样的,是比如现在北京时间20:56 是在这个10分钟内的 做符合阈值判断。 超过阈值的BTCUSDT Large Orders (>10 BTC)
ETHUSDT Large Orders (>500 ETH) 这个标题闪烁
问题1
|
BTCUSDT Large Orders 图表中 两个 BTCUSDT Large Orders (>10 BTC) 改为只要1个,ETHUSDT Orders 图表中 两个 ETHUSDT Large Orders (>500 ETH) 改为只要1个
2024-12-17T21:05:46 改为 2024-12-17 21:05:46 这种格式
Large Orders 有 超过阈值的 不报警,前端不闪烁,邮件看日志有报警了。
024-12-17T21:06:56 Ask 107500.00 112.64
2024-12-17T21:06:57 Bid 3950.00 1501.82
– 1. 创建临时表,包含新的 alert_sent 字段
CREATE TABLE large_orders_temp (
id INTEGER PRIMARY KEY AUTOINCREMENT,
timestamp DATETIME,
symbol TEXT,
price REAL,
quantity REAL,
type TEXT,
current_price REAL,
alert_sent INTEGER DEFAULT 0
);
– 2. 复制现有数据到临时表
INSERT INTO large_orders_temp (timestamp, symbol, price, quantity, type, current_price)
SELECT timestamp, symbol, price, quantity, type, current_price FROM large_orders;
– 3. 删除旧表
DROP TABLE large_orders;
– 4. 重命名新表
ALTER TABLE large_orders_temp RENAME TO large_orders;
1
2
3
4
5
6
7
8
|
---
比如前端页面现在北京时间20:59 是在这个10分钟内的 做符合阈值判断。
超过阈值的,邮件报警,每1个大单只邮件1次报警(一模一样的数据不用在邮件报警),不能重复发。
html 报警的逻辑也是一样的,是比如现在北京时间20:56 是在这个10分钟内的 做符合阈值判断。不管你表alert_sent是否为1 ,只要是当前时间10分钟内的,在前端ETHUSDT BTCUSDT Large Orders 的 超过阈值的 ,就要BTCUSDT Large Orders (>10 BTC)
ETHUSDT Large Orders (>500 ETH) 这个标题闪烁
有看到日志:
|
dict_keys([‘BTCUSDT_107500.0_112.63503_20241217210656’, ‘ETHUSDT_3950.0_1501.8171_20241217210657’])
当前活动报警: set()
….
清理过期报警: BTCUSDT_107500.0_112.63503_20241217210656
清理过期报警: ETHUSDT_3950.0_1501.8171_20241217210657
当前报警状态: dict_keys([])
当前活动报警: set()
1
2
3
4
5
6
7
8
9
10
11
12
13
14
15
16
17
18
19
20
21
22
| 但是前端页面BTCUSDT Large Orders (>10 BTC)
ETHUSDT Large Orders (>500 ETH) 这个标题 没有闪烁
---ok
Price Charts 曲线图表,BTC ETC各图增高15px,其它不变
1:现在去30秒去获取ask bid 单子。是在哪里定义的。能否统一写到config定义
--- ok
schedule.every(30).seconds.do(fetch_and_process_data)。这个30 能在写到config定义吗
---
--ls
2:前端页面 页面的最底部靠右边 往上高32px 宽为1000px宽度区域。从右边往左滚动字幕显示 lizhi.txt文件的1行文字,(这个文件多行文件记录,从上往下循环)。
:在 BTCUSDT Large Orders (>10 BTC) ETHUSDT Large Orders (>500 ETH)的下面。
--- ls
|
Time Range: 0h-24h,这个是指具体哪个值的范围, 现在代码默认范围选择的是多少?
在 display.py 中的 Time Range 滑块是用于时间范围选择的,目前默认值是 [23, 24],也就是最后一个小时:
dcc.RangeSlider(
id=‘time-range-slider’,
min=0,
max=24,
step=1,
marks={i: f’{i}h’ for i in range(0, 25, 4)},
value=[23, 24] # 默认选择最后一个小时
)```
这个时间范围应该是用来过滤订单数据的,但我看代码中并没有使用这个滑块的值。我们应该在查询数据时使用这个时间范围。
3 : Update Interval:30 seconds 这个下拉选择框能放在 Time Range:的后面吗? Update Interval:30 seconds 这个下拉选择框高35px 宽110px ,跟Time Range水平齐平显示 ,Time Range 宽850 高35px。
Price Chart Timeframe 下拉选择框高35px 宽110px ,靠右边靠齐 ,也跟Time Range水平齐平显示 。 你现在文字Update Interval:、 Price Chart Timeframe: 和下拉框都要有啊。
1
2
3
4
5
6
7
8
9
10
11
12
13
14
15
16
17
18
19
20
21
22
23
| ERROR:__main__:程序启动时发生错误: The `dcc.RangeSlider` component (version 2.18.2) with the ID "time-range-slider" received an unexpected keyword argument: `style`
Allowed arguments: allowCross, className, count, disabled, dots, drag_value, id, included, loading_state, marks, max, min, persisted_props, persistence, persistence_type, pushable, step, tooltip, updatemode, value, vertical, verticalHeight
ERROR:__main__:Traceback (most recent call last):
File "E:\act_now\IncrementalSteps\bid_ask\main.py", line 123, in main
app = create_app()
^^^^^^^^^^^^
File "E:\act_now\IncrementalSteps\bid_ask\main.py", line 26, in create_app
display = CryptoDisplay()
^^^^^^^^^^^^^^^
File "E:\act_now\IncrementalSteps\bid_ask\bid_ask\display.py", line 12, in __init__
self.layout = self.create_layout()
^^^^^^^^^^^^^^^^^^^^
File "E:\act_now\IncrementalSteps\bid_ask\bid_ask\display.py", line 25, in create_layout
dcc.RangeSlider(
File "C:\Users\Administrator\AppData\Roaming\Python\Python311\site-packages\dash\development\base_component.py", line 446, in wrapper
return func(*args, **kwargs)
^^^^^^^^^^^^^^^^^^^^^
File "C:\Users\Administrator\AppData\Roaming\Python\Python311\site-packages\dash\dcc\RangeSlider.py", line 239, in __init__
super(RangeSlider, self).__init__(**args)
File "C:\Users\Administrator\AppData\Roaming\Python\Python311\site-packages\dash\development\base_component.py", line 157, in __init__
raise TypeError(
TypeError: The `dcc.RangeSlider` component (version 2.18.2) with the ID "time-range-slider" received an unexpected keyword argument: `style`
Allowed arguments: allowCross, className, count, disabled, dots, drag_value, id, included, loading_state, marks, max, min, persisted_props, persistence, persistence_type, pushable, step, tooltip, updatemode, value, vertical, verticalHeight
|
BTCUSDT Large Orders (>10 BTC) 或者 ETHUSDT Large Orders (>500 ETH)
有个小细节,Time列头和 下面数据比如 2024-12-17 22:58:39 不整齐,看起来有点点乱 ,每列文字居中显示。这样列头和下面的数据能做到整齐吗。 或者用你知道的方式。
BTCUSDT Large Orders (>10 BTC) 或者 ETHUSDT Large Orders (>500 ETH) 有2个下滑框感觉不美观。 只要里面订单那个下滑框。
改下BTCUSDT Large Orders (>10 BTC) 和 ETHUSDT Large Orders (>500 ETH) 的高度 改为32px ;列头高25px。 数据每行高32px
为什么ETHUSDT的Depth Analysis 显示为Depth Analysis: near:999.99 mid:999.99 far:999.99 这不正常吧?
为什么ETHUSDT和BTCUSDT的Depth Analysis 都显示为Depth Analysis: near:2 mid:2 far:2 这正常吗? 如果都为2 代表什么?
ETHUSDT的趋势注释 显示为 strong buliish B/A Ratio:6.88 .这说明啥? 其它参数有哪些呢?
为什么 ETHUSDT BTCUSDT的买卖强度图中的时间显示 2024 12/17 07:54:30,转换为北京时间显示。
1
2
3
4
5
6
7
8
9
10
11
12
13
14
15
16
17
18
19
20
21
22
23
24
25
26
| Traceback (most recent call last):
File "E:\act_now\IncrementalSteps\bid_ask\main.py", line 1, in <module>
from bid_ask.data_fetcher import CryptoDataFetcher
File "E:\act_now\IncrementalSteps\bid_ask\bid_ask\data_fetcher.py", line 5, in <module>
from binance.client import Client
File "C:\Users\Administrator\AppData\Roaming\Python\Python311\site-packages\binance\__init__.py", line 9, in <module>
from binance.async_client import AsyncClient # noqa
^^^^^^^^^^^^^^^^^^^^^^^^^^^^^^^^^^^^^^^^^^^^
File "C:\Users\Administrator\AppData\Roaming\Python\Python311\site-packages\binance\async_client.py", line 21, in <module>
from .base_client import BaseClient
File "C:\Users\Administrator\AppData\Roaming\Python\Python311\site-packages\binance\base_client.py", line 10, in <module>
from Crypto.PublicKey import RSA, ECC
File "C:\Users\Administrator\AppData\Roaming\Python\Python311\site-packages\Crypto\PublicKey\RSA.py", line 43, in <module>
from Crypto.Math.Numbers import Integer
File "C:\Users\Administrator\AppData\Roaming\Python\Python311\site-packages\Crypto\Math\Numbers.py", line 39, in <module>
from Crypto.Math._IntegerGMP import IntegerGMP as Integer
File "C:\Users\Administrator\AppData\Roaming\Python\Python311\site-packages\Crypto\Math\_IntegerGMP.py", line 36, in <module>
from Crypto.Util._raw_api import (backend, load_lib,
File "C:\Users\Administrator\AppData\Roaming\Python\Python311\site-packages\Crypto\Util\_raw_api.py", line 87, in <module>
ffi = FFI()
^^^^^
File "C:\Users\Administrator\AppData\Roaming\Python\Python311\site-packages\cffi\api.py", line 54, in __init__
raise Exception("Version mismatch: this is the 'cffi' package version %s, located in %r. When we import the top-level '_cffi_backend' extension module, we get version %s, located in %r. The two versions should be equal; check your installation." % (
Exception: Version mismatch: this is the 'cffi' package version 1.17.1, located in 'C:\\Users\\Administrator\\AppData\\Roaming\\Python\\Python311\\site-packages\\cffi\\api.py'. When we import the top-level '_cffi_backend' extension module, we get version 1.16.0, located in 'D:\\Software\\miniconda3\\Lib\\site-packages\\_cffi_backend.cp311-win_amd64.pyd'. The two versions should be equal; check your installation.
进程已结束,退出代码为 1
|
1
2
3
4
5
6
7
8
9
10
11
12
13
14
15
16
17
18
19
20
21
22
23
24
25
26
27
28
29
30
31
32
33
34
35
36
37
38
39
40
41
42
43
44
45
46
47
48
49
50
51
52
53
54
55
56
57
58
59
60
61
62
63
64
65
66
67
68
69
70
71
72
73
74
75
76
77
78
79
80
81
82
83
84
85
86
87
88
89
90
91
92
93
94
95
96
97
98
99
100
101
102
103
104
105
106
107
108
109
110
111
112
113
114
115
116
117
118
|
import pandas as pd
import numpy as np
from binance.client import Client
from binance import Client, BinanceSocketManager
from datetime import datetime, timedelta
# Binance API设置
api_key = '5nV9ChLDDCSgIPkNWpBrKJlKQOMdIleOjSdYp1gvvRCAaF5L9gZtRx20RM8CMxQ8'
api_secret = '5xHbdWenlYBF8FwU4FtiKQfjcUl8EG3XmIpmuEmajptVoZe7tyxBMTZmblIrrO0K'
client = Client(api_key, api_secret)
def fetch_1day_data(symbol):
"""
从Binance获取当前时间之前一天的K线数据。
参数:
symbol - 交易对符号,例如'ETHUSDT'
返回:
pandas DataFrame - 包含一天的数据
"""
now = datetime.utcnow()
start = now - timedelta(days=1)
end = now
# 获取1小时K线数据
klines = client.get_historical_klines(symbol, Client.KLINE_INTERVAL_1HOUR, start.strftime("%d %b %Y %H:%M:%S"), end.strftime("%d %b %Y %H:%M:%S"))
# 转换数据到DataFrame
df = pd.DataFrame(klines, columns=['timestamp', 'open', 'high', 'low', 'close', 'volume', 'close_time', 'quote_asset_volume', 'number_of_trades', 'taker_buy_base_asset_volume', 'taker_buy_quote_asset_volume', 'ignore'])
df['timestamp'] = pd.to_datetime(df['timestamp'], unit='ms')
df = df.set_index('timestamp')
df = df[['open', 'high', 'low', 'close', 'volume']].astype(float)
return df
def grid_strategy_daily(data, total_capital, fee_rate=0.001):
"""
基于一天的数据确定网格交易策略的参数。
参数:
data - pandas DataFrame,包含日期和价格数据
total_capital - 总投资资本
fee_rate - 交易手续费率,默认0.1%(0.001)
返回:
dict - 包含策略参数和预期收益的字典
"""
# 计算一天中的最高价和最低价
high = data['high'].max()
low = data['low'].min()
# 计算区间上下限(这里假设上下限为当天最高价和最低价的1.1倍和0.9倍)
upper_limit = high * 1.1
lower_limit = low * 0.9
# 计算网格间隔(假设为当天价格波动范围的1%)
price_range = high - low
grid_interval = price_range * 0.01
# 计算交易数量(假设每次交易使用总资本的2%)
trade_quantity = total_capital * 0.02 / high # 使用最高价计算数量以确保安全
# 模拟交易
trades = []
for i in range(len(data)):
if i == 0: # 第一天不交易
continue
# 买入条件
if data['close'].iloc[i] < data['close'].iloc[i-1] and data['close'].iloc[i] < upper_limit:
if data['close'].iloc[i] > lower_limit + grid_interval: # 确保不在最低价买入
buy_price = data['close'].iloc[i]
trades.append({'type': 'buy', 'price': buy_price, 'quantity': trade_quantity})
# 卖出条件
elif data['close'].iloc[i] > data['close'].iloc[i-1] and data['close'].iloc[i] > lower_limit:
if data['close'].iloc[i] < upper_limit - grid_interval: # 确保不在最高价卖出
sell_price = data['close'].iloc[i]
trades.append({'type': 'sell', 'price': sell_price, 'quantity': trade_quantity})
# 计算交易成本和收益
total_fee = 0
total_profit = 0
for trade in trades:
if trade['type'] == 'buy':
total_fee += trade['price'] * trade['quantity'] * fee_rate
elif trade['type'] == 'sell':
total_fee += trade['price'] * trade['quantity'] * fee_rate
# 假设卖出价格为买入价格加一个网格间隔的价格差
profit = (trade['price'] - (trade['price'] - grid_interval)) * trade['quantity'] - 2 * (trade['price'] * trade['quantity'] * fee_rate)
total_profit += profit
# 计算总成本(这里只计算交易费用)
total_cost = total_fee
# 返回结果
return {
'upper_limit': upper_limit,
'lower_limit': lower_limit,
'grid_interval': grid_interval,
'trade_quantity': trade_quantity,
'total_cost': total_cost,
'total_profit': total_profit,
'net_profit': total_profit - total_cost,
'trades': trades
}
# 使用示例
symbol = 'ETHUSDT'
total_capital = 10000 # 假设总资本为10000美元
# 获取最近一天的数据
data = fetch_1day_data(symbol)
# 计算网格交易策略
result = grid_strategy_daily(data, total_capital)
print(result)
|
1
2
3
4
5
6
7
8
9
10
11
12
13
14
15
16
17
18
19
20
21
22
23
24
25
26
27
28
29
30
31
32
33
34
35
36
37
38
39
40
41
42
43
44
45
46
47
48
49
50
51
52
53
54
55
56
57
58
59
60
61
62
63
64
65
66
67
68
69
70
71
72
73
74
75
76
77
| main.py 程序 不用nginx 可以直接通过 访问 http://127.0.0.1:8050/是可以访问的。
现在 做了nginx代理 通过 www.test.com/bid_ask/访问不了。
代理配置如下
location /bid_ask/ {
proxy_pass http://127.0.0.1:8050/;
proxy_set_header Host $host;
proxy_set_header X-Real-IP $remote_addr;
proxy_set_header X-Forwarded-For $proxy_add_x_forwarded_for;
proxy_set_header X-Forwarded-Proto $scheme;
}
F12 发现这些
<script src="/_dash-component-suites/dash/deps/polyfill@7.v2_18_2m1734702683.12.1.min.js"></script>
<script src="/_dash-component-suites/dash/deps/react@16.v2_18_2m1734702683.14.0.js"></script>
<script src="/_dash-component-suites/dash/deps/react-dom@16.v2_18_2m1734702683.14.0.js"></script>
<script src="/_dash-component-suites/dash/deps/prop-types@15.v2_18_2m1734702683.8.1.js"></script>
<script src="/_dash-component-suites/dash/dash-renderer/build/dash_renderer.v2_18_2m1734702682.dev.js"></script>
<script src="/_dash-component-suites/dash/dcc/dash_core_components.v2_14_3m1734702683.js"></script>
<script src="/_dash-component-suites/dash/dcc/dash_core_components-shared.v2_14_3m1734702683.js"></script>
<script src="/_dash-component-suites/dash/html/dash_html_components.v2_0_20m1734702683.min.js"></script>
<script src="/_dash-component-suites/dash/dash_table/bundle.v5_2_13m1734702682.js"></script>
https://www.test.com/_dash-component-suites/dash/dash_table/bundle.v5_2_13m1734702682.js 报404
https://www.test.com/bid_ask/_dash-component-suites/dash/dash_table/bundle.v5_2_13m1734702682.js 这样是可以访问的。
如何不改程序的情况下或这好多方式。 解决这个问题。
location /_dash-component-suites/ {
proxy_pass http://127.0.0.1:8050/_dash-component-suites/;
现在https://www.test.com/_dash-component-suites/dash/dash_table/bundle.v5_2_13m1734702682.js 可以了
但现在 https://www.test.com/_reload-hash 报404 了
---
https://www.2urs.com/_dash-component-suites/dash/dash_table/bundle.v5_2_13m1734702682.js 404
https://www.2urs.com/_reload-hash 404
----
location /bid_ask/ {
proxy_pass http://127.0.0.1:8050/;
proxy_set_header Host $host;
proxy_set_header X-Real-IP $remote_addr;
proxy_set_header X-Forwarded-For $proxy_add_x_forwarded_for;
proxy_set_header X-Forwarded-Proto $scheme;
}
location /_dash-component-suites/ {
proxy_pass http://127.0.0.1:8050/_dash-component-suites/;
proxy_set_header Host $host;
proxy_set_header X-Real-IP $remote_addr;
proxy_set_header X-Forwarded-For $proxy_add_x_forwarded_for;
proxy_set_header X-Forwarded-Proto $scheme;
}
##
# 添加处理 _reload-hash 的路由
location /_reload-hash {
proxy_pass http://127.0.0.1:8050/_reload-hash;
proxy_set_header Host $host;
proxy_set_header X-Real-IP $remote_addr;
proxy_set_header X-Forwarded-For $proxy_add_x_forwarded_for;
proxy_set_header X-Forwarded-Proto $scheme;
}
# 处理其他 Dash 相关的路径
location ~ ^/_dash-.*$ {
proxy_pass http://127.0.0.1:8050$request_uri;
proxy_set_header Host $host;
proxy_set_header X-Real-IP $remote_addr;
proxy_set_header X-Forwarded-For $proxy_add_x_forwarded_for;
proxy_set_header X-Forwarded-Proto $scheme;
}
ok
|
python binance实现网格交易自动交易:
首先要制定一个基于最近2天的K线数据来确定现阶段 网格最合理的交易策略的区间上下限、网格间隔和交易数量,并计算成本与获益的函数
自动获取当前时间为止2天的数据。通过你的函数 确定现阶段 网格最合理的交易策略的区间上下限、网格间隔和交易数量,并计算成本与获益【你可以给3组数据 】。使使用者能知道哪个区间上下限、网格间隔和交易数量,并计算成本与获益 最合适。获益最大,
并且少亏损 甚至自动止损。
ccxt与binance的库有什么区别。如果要做自动交易系统哪个好?
eth最高价格是多少 这段时间发生了什么 为什么有这么高的价格,同期btc的价格是多少?
用户: 【鲸鱼 灰度 @ai_9684xtpa 巨鲸 新地址0x148…640c8】
动作: 建仓 买入 减仓 提出 卖出 加仓
时间: 北京时间
货币: BTC ETH ETF DOGE LINK
数量: 2894枚 1000个 10%仓位
买入价格: 成本3681美元 买入价格3821美元 成本3681 买价3821 建仓价格3821
卖出价格: 现价3981美元 卖出价格3821美元 卖价3881美元 卖出价格3821美元
亏盈: 浮盈113万美元 亏299美元
波段胜率 70%